Helping ARX, a cyber-security FinTech with interim COO services to scale-up their delivery
We were engaged by ARX to provide an interim COO as they gaining traction in the market and needed to scale their operations to support their new clients. We used our financial services delivery experience to take on UX/UI design, redesign their operational processes for scale, and be a delivery partner for their supply chain resilience solution.
Due to our efforts, ARX were able to meet their client demand with an improved product and more efficient sales & go-to-market approach.
Increasing data product offerings by profiling 80k terms at a global data provider
“Through domain & technical expertise Leading Point have been instrumental in the success of this project to analyse and remediate 80k industry terms. LP have developed a sustainable process, backed up by technical tools, allowing the client to continue making progress well into the future. I would have no hesitation recommending LP as a delivery partner to any firm who needs help untangling their data.”
PM at Global Market Data Provider
AI in Insurance - Article 1 - A Catalyst for Innovation
How insurance companies can use the latest AI developments to innovate their operations
The emergence of AI
The insurance industry is undergoing a profound transformation driven by the relentless advance of artificial intelligence (AI) and other disruptive technologies. A significant change in business thinking is gaining pace and Applied AI is being recognised for its potential in driving top-line growth and not merely a cost-cutting tool.
The adoption of AI is poised to reshape the insurance industry, enhancing operational efficiencies, improving decision-making, anticipating challenges, delivering innovative solutions, and transforming customer experiences.
This shift from data-driven to AI-driven operations is bringing about a paradigm shift in how insurance companies collect, analyse, and utilise data to make informed decisions and enhance customer experiences. By analysing vast amounts of data, including historical claims records, market forces, and external factors (global events like hurricanes, and regional conflicts), AI can assess risk with speed and accuracy to provide insurance companies a view of their state of play in the market.
Data vs AI approaches
This data-driven approach has enabled insurance companies to improve their underwriting accuracy, optimise pricing models, and tailor products to specific customer needs. However, the limitations of traditional data analytics methods have become increasingly apparent in recent years.
These methods often struggle to capture the complex relationships and hidden patterns within large datasets. They are also slow to adapt to rapidly-changing market conditions and emerging risks. As a result, insurance companies are increasingly turning to AI to unlock the full potential of their data and drive innovation across the industry.
AI algorithms, powered by machine learning and deep learning techniques, can process vast amounts of data far more efficiently and accurately than traditional methods. They can connect disparate datasets, identify subtle patterns, correlations & anomalies that would be difficult or impossible to detect with human analysis.
By leveraging AI, insurance companies can gain deeper insights into customer behaviour, risk factors, and market trends. This enables them to make more informed decisions about underwriting, pricing, product development, and customer service and gain a competitive edge in the ever-evolving marketplace.
Top 5 opportunities
1. Enhanced Risk Assessment
AI algorithms can analyse a broader range of data sources, including social media posts and weather patterns, to provide more accurate risk assessments. This can lead to better pricing and reduced losses.
2. Personalised Customer Experiences
AI can create personalised customer experiences, from tailored product recommendations to proactive risk mitigation guidance. This can boost customer satisfaction and loyalty.
3. Automated Claims Processing
AI can automate routine claims processing tasks, for example, by reviewing claims documentation and providing investigation recommendations, thus reducing manual efforts and improving efficiency. This can lead to faster claims settlements and lower operating costs.
4. Fraud Detection and Prevention
AI algorithms can identify anomalies and patterns in claims data to detect and prevent fraudulent activities. This can protect insurance companies from financial losses and reputational damage.
5. Predictive Analytics
AI can be used to anticipate future events, such as customer churn or potential fraud. This enables insurance companies to take proactive measures to prevent negative outcomes.
Adopting AI in Insurance
The adoption of AI in the insurance industry is not without its challenges. Insurance companies must address concerns about data quality, data privacy, transparency, and potential biases in AI algorithms. They must also ensure that AI is integrated seamlessly into their existing systems and processes.
Despite these challenges, AI presents immense opportunities. Insurance companies that embrace AI-driven operations will be well-positioned to gain a competitive edge, enhance customer experiences, and navigate the ever-changing risk landscape.
The shift from data-driven to AI-driven operations is a transformative force in the insurance industry. AI is not just a tool for analysing data; it is a catalyst for innovation and a driver of change. Insurance companies that harness the power of AI will be at the forefront of this transformation, shaping the future of insurance and delivering exceptional value to their customers.
Download the PDF article here.
The Challenges of Data Management
John Macpherson on The Challenges of Data Management
I often get asked, what are the biggest trends impacting the Financial Services industry? Through my position as Chair of the Investment Association Engine, I have unprecedented access to the key decision-makers in the industry, as well as constant connectivity with the ever-expanding Fintech ecosystem, which has helped me stay at the cutting edge of the latest trends.
So, when I get asked, ‘what is the biggest trend that financial services will face’, for the past few years my answer has remained the same, data.
During my time as CEO of BMLL, big data rose to prominence and developed into a multi-billion-dollar problem across financial services. I remember well an early morning interview I gave to CNBC around 5 years ago, where the facts were starkly presented. Back then, data was doubling every three years globally, but at an even faster pace in financial markets.
Firms are struggling under the weight of this data
The use of data is fundamental to a company's operations, but they are finding it difficult to get a handle on this problem. The pace of this increase has left many smaller and mid-sized IM/ AM firms in a quandary. Their ability to access, manage and use multiple data sources alongside their own data, market data, and any alternative data sources, is sub-optimal at best. Most core data systems are not architected to address the volume and pace of change required, with manual reviews and inputs creating unnecessary bottlenecks. These issues, among a host of others, mean risk management systems cannot cope as a result. Modernised data core systems are imperative to solve where real-time insights are currently lost, with fragmented and slow-moving information.
Around half of all financial service data goes unmentioned and ungoverned, this “dark data” poses a security and regulatory risk, as well as a huge opportunity.
While data analytics, big data, AI, and data science are historically the key sub-trends, these have been joined by data fabric (as an industry standard), analytical ops, data democratisation, and a shift from big data to smaller and wider data.
Operating models hold the key to data management
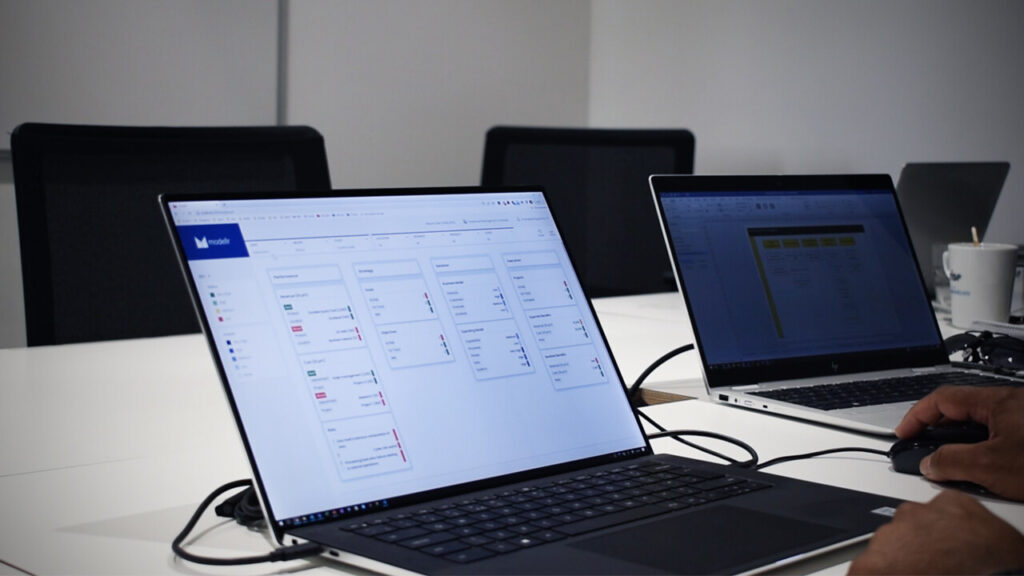
Governance is paramount to using this data in an effective, timely, accurate and meaningful way. Operating models are the true gauge as to whether you are succeeding.
Much can be achieved with the relatively modest budget and resources firms have, provided they invest in the best operating models around their data.
Leading Point is a firm I have been getting to know over several years now. Their data intelligence platform modellr™, is the first truly digital operating model. modellr™ harvests a company’s existing data to create a living operating model, digitising the change process, and enabling quicker, smarter, decision making. By digitising the process, they’re removing the historically slow and laborious consultative approach. Access to all the information in real-time is proving transformative for smaller and medium-sized businesses.
True transparency around your data, understanding it and its consumption, and then enabling data products to support internal and external use cases, is very much available.
Different firms are at very different places on their maturity curve. Longer-term investment in data architecture, be it data fabric or data mesh, will provide the technical backbone to harvest ML/ AI and analytics.
Taking control of your data
Recently I was talking to a large investment bank for whom Leading Point had been brought in to help. The bank was looking to transform its client data management and associated regulatory processes such as KYC, and Anti-financial crime.
They were investing heavily in sourcing, validating, normalising, remediating, and distributing over 2,000 data attributes. This was costing the bank a huge amount of time, money, and resources. But, despite the changes, their environment and change processes had become too complicated to have any chance of success. The process results were haphazard, with poor controls and no understanding of the results missing.
Leading Point was brought in to help and decided on a data minimisation approach. They profiled and analysed the data, despite working across regions and divisions. Quickly, 2,000 data attributes were narrowed to less than 200 critical ones for the consuming functions. This allowed the financial institutions, regulatory, and reporting processes to come to life, with clear data quality measurement and ownership processes. It allowed the financial institutions to significantly reduce the complexity of their data and its usability, meaning that multiple business owners were able to produce rapid and tangible results
I was speaking to Rajen Madan, the CEO of Leading Point, and we agreed that in a world of ever-growing data, data minimisation is often key to maximising success with data!
Elsewhere, Leading Point has seen benefits unlocked from unifying data models, and working on ontologies, standards, and taxonomies. Their platform, modellr™is enabling many firms to link their data, define common aggregations, and support knowledge graph initiatives allowing firms to deliver more timely, accurate and complete reporting, as well as insights on their business processes.
The need for agile, scalable, secure, and resilient tech infrastructure is more imperative than ever. Firms’ own legacy ways of handling this data are singularly the biggest barrier to their growth and technological innovation.
If you see a digital operating model as anything other than a must-have, then you are missing out. It’s time for a serious re-think.
Words by John Macpherson — Board advisor at Leading Point, Chair of the Investment Association Engine
John was recently interviewed about his role at Leading Point, and the key trends he sees affecting the financial services industry. Watch his interview here
Artificial Intelligence: The Solution to the ESG Data Gap?
The Power of ESG Data
It was Warren Buffett who said, “It takes twenty years to build a reputation and five minutes to ruin it” and that is the reality that all companies face on a daily basis. An effective set of ESG (Environment, Social & Governance) policies has never been more crucial. However, it is being hindered by difficulties surrounding the effective collection and communication of ESG data points, as well a lack of standardisation when it comes to reporting such data. As a result, the ESG space is being revolutionised by Artificial Intelligence, which can find, analyse and summarise this information.
There is increasing public and regulatory pressure on firms to ensure their policies are sustainable and on investors to take such policies into account when making investment decisions. The issue for investors is how to know which firms are good ESG performers and which are not. The majority of information dominating research and ESG indices comes from company-reported data. However, with little regulation surrounding this, responsible investors are plagued by unhelpful data gaps and “Greenwashing”. This is when a firm uses favourable data points and convoluted wording to appear more sustainable than they are in reality. They may even leave out data points that reflect badly on them. For example, firms such as Shell are accused of using the word ‘sustainable’ in their mission statement whilst providing little evidence to support their claims (1).
Could AI be the complete solution?
AI could be the key to help investors analyse the mountain of ESG data that is yet to be explored, both structured and unstructured. Historically, AI has been proven to successfully extract relevant information from data sources including news articles but it also offers new and exciting opportunities. Consider the transcripts of board meetings from a Korean firm: AI could be used to translate and examine such data using techniques such as Sentiment Analysis. Does the CEO seem passionate about ESG issues within the company? Are they worried about an investigation into Human Rights being undertaken against them? This is a task that would be labour-intensive, to say the least, for analysts to complete manually.
In addition, AI offers an opportunity for investors to not only act responsibly, but also align their ESG goals to a profitable agenda. For example, algorithms are being developed that can connect specific ESG indicators to financial performance and can therefore be used by firms to identify the risk and reward of certain investments.
Whilst AI offers numerous opportunities with regards to ESG investing, it is not without fault. Firstly, AI takes enormous amounts of computing power and, hence, energy. For example, in 2018, OpenAI found the level of computational power used to train the largest AI models has been doubling every 3.4 months since 2012 (2). With the majority of the world’s energy coming from non-renewable sources, it is not difficult to spot the contradiction in motives here. We must also consider whether AI is being used to its full potential; when simply used to scan company published data, AI could actually reinforce issues such as “Greenwashing”. Further, the issue of fake news and unreliable sources of information still plagues such methods and a lot of work has to go into ensuring these sources do not feature in algorithms used.
When speaking with Dr Thomas Kuh, Head of Index at leading ESG data and AI firm Truvalue Labs™, he outlined the difficulties surrounding AI but noted that since it enables human beings to make more intelligent decisions, it is surely worth having in the investment process. In fact, he described the application of AI to ESG research as ‘inevitable’ as long as it is used effectively to overcome the shortcomings of current research methods. For instance, he emphasised that AI offers real time information that traditional sources simply cannot compete with.
A Future for AI?
According to a 2018 survey from Greenwich Associates (3), only 17% of investment professionals currently use AI as part of their process; however, 40% of respondents stated they would increase budgets for AI in the future. As an area where investors are seemingly unsatisfied with traditional data sources, ESG is likely to see more than its fair share of this increase. Firms such as BNP Paribas (4) and Ecofi Investissements (5) are already exploring AI opportunities and many firms are following suit. We at Leading Point see AI inevitably becoming integral to an effective responsible investment process and intend to be at the heart of this revolution.
AI is by no means the judge, jury and executioner when it comes to ESG investing and depends on those behind it, constantly working to improve the algorithms, as well as the analysts using it to make more informed decisions. AI does, however, have the potential to revolutionise what a responsible investment means and help reallocate resources towards firms that will create a better future.
[1] The problem with corporate greenwashing
[2] AI and Compute
[3] Could AI Displace Investment Bank Research?
[4] How AI could shape the future of investment banking
[5] How AI Can Help Find ESG Opportunities
"It takes twenty years to build a reputation and five minutes to ruin it"
AI offers an opportunity for investors to not only act responsibly, but also align their ESG goals to a profitable agenda
Environmental Social Governance (ESG) & Sustainable Investment
Client propositions and products in data driven transformation in ESG and Sustainable Investing. Previous roles include J.P. Morgan, Morgan Stanley, and EY.
Upcoming blogs:
This is the second in a series of blogs that will explore the ESG world: its growth, its potential opportunities and the constraints that are holding it back. We will explore the increasing importance of ESG and how it affects business leaders, investors, asset managers, regulatory actors and more.
Riding the ESG Regulatory Wave: In the third part of our Environmental, Social and Governance (ESG) blog series, Alejandra explores the implementation challenges of ESG regulations hitting EU Asset Managers and Financial Institutions.
Is it time for VCs to take ESG seriously? In the fourth part of our Environmental, Social and Governance (ESG) blog series, Ben explores the current research on why startups should start implementing and communicating ESG policies at the core of their business.
Now more than ever, businesses are understanding the importance of having well-governed and socially-responsible practices in place. A clear understanding of your ESG metrics is pivotal in order to communicate your ESG strengths to investors, clients and potential employees.
By using our cloud-based data visualisation platform to bring together relevant metrics, we help organisations gain a standardised view and improve your ESG reporting and portfolio performance. Our live ESG dashboard can be used to scenario plan, map out ESG strategy and tell the ESG story to stakeholders.
AI helps with the process of ingesting, analysing and distributing data as well as offering predictive abilities and assessing trends in the ESG space. Leading Point is helping our AI startup partnerships adapt their technology to pursue this new opportunity, implementing these solutions into investment firms and supporting them with the use of the technology and data management.
We offer a specialised and personalised service based on firms’ ESG priorities. We harness the power of technology and AI to bridge the ESG data gap, avoiding ‘greenwashing’ data trends and providing a complete solution for organisations.
Leading Point's AI-implemented solutions decrease the time and effort needed to monitor current/past scandals of potential investments. Clients can see the benefits of increased output, improved KPIs and production of enhanced data outputs.
Implementing ESG regulations and providing operational support to improve ESG metrics for banks and other financial institutions. Ensuring compliance by benchmarking and disclosing ESG information, in-depth data collection to satisfy corporate reporting requirements, conducting appropriate investment and risk management decisions, and to make disclosures to clients and fund investors.
LIBOR Transition - Preparation in the Face of Adversity
LIBOR TRANSITION IN CONTEXT
What is it? FCA will no longer seek require banks to submit quotes to the London Interbank Offered Rate (LIBOR) – LIBOR will be unsupported by regulators come 2021, and therefore, unreliable
Requirement: Firms need to transition away from LIBOR to alternative overnight risk-free rates (RFRs)
Challenge: Updating the risk and valuation processes to reflect RFR benchmarks and then reviewing the millions of legacy contracts to remove references to IBOR
Implementation timeline: Expected in Q4 2021
HOW LIBOR MAY IMPACT YOUR BUSINESS
Front office: New issuance and trading products to support capital, funding, liquidity, pricing, hedging
Finance & Treasury: Balance sheet valuation and accounting, asset, liability and liquidity management
Risk Management: New margin, exposure, counterparty risk models, VaR, time series, stress and sensitivities
Client outreach: Identification of in-scope contracts, client outreach and repapering to renegotiate current exposure
Change management: F2B data and platform changes to support all of the above
WHAT YOU NEED TO DO
Plug in to the relevant RFR and trade association working groups, understand internal advocacy positions vs. discussion outcomes
Assess, quantify and report LIBOR exposure across jurisdictions, businesses and products
Remediate data quality and align product taxonomies to ensure integrity of LIBOR exposure reporting
Evaluate potential changes to risk and valuation models; differences in accounting treatment under an alternative RFR regime
Define list of in-scope contracts and their repapering approach; prepare for client outreach
“[Firms should be] moving to contracts which do not rely on LIBOR and will not switch references rates at an unpredictable time”
Andrew Bailey, CEO,
Financial Conduct Authority (FCA)
“Identification of areas of no-regret spending is critical in this initial phase of delivery so as to give a head start to implementation”
Rajen Madan, CEO,
Leading Point FM
BENCHMARK TRANSITION KEY FACTS
- Market Exposure - Total IBOR market exposure >$370TN 80% represented by USD LIBOR & EURIBOR
- Tenor - The 3-month tenor by volume is the most widely referenced rate in all currencies (followed by the 6-month tenor)
- Derivatives - OTC and exchange traded derivatives represent > $300TN (80%) of products referencing IBORs
- Syndicated Loans - 97% of syndicated loans in the US market, with outstanding volume of approximately $3.4TN, reference USD LIBOR. 90% of syndicated loans in the euro market, with outstanding volume of approximately $535BN, reference EURIBOR
- Floating Rate Notes (FRNs) - 84% of FRNs inthe US market, with outstanding volume of approximately $1.5TN, reference USD LIBOR. 70% of FRNs in the euro market,with outstanding volume of approximately $2.6TN, reference EURIBOR
- Business Loans - 30%-50% of business loans in the US market, with outstanding volume of approximately $2.9TN, reference USD LIBOR. 60% of business loans in the euro market, with outstanding volume of approximately $5.8TN, reference EURIBOR
*(“IBOR Global Benchmark Survey 2018 Transition Roadmap”, ISDA, AFME, ICMA, SIFMA, SIFMA AM, February 2018)
Data Innovation, Uncovered
Leading Point Financial Markets recently partnered with selected tech companies to present innovative solutions to a panel of SMEs and an audience of FS senior execs and practitioners across 5 use-cases Leading Point is helping financial institutions with. The panel undertook a detailed discussion on the solutions’ feasibility within these use-cases, and their potential for firms, followed by a lively debate between Panellists and Attendees.
EXECUTIVE SUMMARY
“There is an opportunity to connect multiple innovation solutions to solve different, but related, business problems”
- 80% of data is relatively untapped in organisations. The more familiar the datasets, the better data can be used
- On average, an estimated £84 million (expected to be a gross underestimation) is wasted each year from increasing risk and delivery from policies and regulations
- Staying innovative, while staying true to privacy data is a fine line. Solutions exist in the marketplace to help
- Is there effective alignment between business and IT? Panellists insisted there is a significantly big gap, but using business architecture can be a successful bridge between the business and IT, by driving the right kinds of change
- There is a huge opportunity to blend these solutions to provide even more business benefits
CLIENT DATA LIFECYCLE (TAMR)
- Tamr uses machine learning to combine, consolidate and classify disparate data sources with potential to improve customer segmentation analytics
- To achieve the objective of a 360-degree view of the customer requires merging external datasets with internal in a appropriate and efficient manner, for example integrating ‘Politically Exposed Persons’ lists or sanctions ‘blacklists’
- Knowing what ‘good’ looks like is a key challenge. This requires defining your comfort level, in terms of precision and probability based approaches, versus the amount of resource required to achieve those levels
- Another challenge is convincing Compliance that machines are more accurate than individuals
- To convince the regulators, it is important to demonstrate that you are taking a ‘joined up’ approach across customers, transactions, etc. and the rationale behind that approach
LEGAL DOCS TO DATA (iManage)
- iManage locates, categorises & creates value from all your contractual content
- Firms hold a vast amount of legal information in unstructured formats - Classifying 30,000,000 litigation documents manually would take 27 years
- However, analysing this unstructured data and converting it to structured digital data allows firms to conduct analysis and repapering exercises with much more efficiency
- It is possible to a) codify regulations & obligations b) compare them as they change and c) link them to company policies & contracts – this enables complete traceability
- For example, you can use AI to identify parties, dates, clauses & conclusions held within ISDA contract forms, reports, loan application contracts, accounts and opinion pieces
DATA GOVERNANCE (Io-Tahoe)
- Io-Tahoe LLC is a provider of ‘smart’ data discovery solutions that go beyond traditional metadata and leverages machine learning and AI to look at implied critical and often unknown relationships within the data itself
- Io-Tahoe interrogates any structured/semi-structured data (both schema and underlying data) and identifies and classifies related data elements to determine their business criticality
- Pockets of previously-hidden sensitive data can be uncovered enabling better compliance to data protection regulations, such as GDPR
- Any and all data analysis is performed on copies of the data held wherever the information security teams of the client firms deems it safe
- Once data elements are understood, they can be defined & managed and used to drive data governance management processes
FINANCIAL CRIME (Ayasdi)
- Ayasdi augments the AML process with intelligent segmentation, typologies and alert triage. Their topological data analysis capabilities provide a formalised and repeatable way of applying hundreds of combinations of different machine learning algorithms to a data set to find out the relationships within that data
- For example, Ayasdi was used reason-based elements in predictive models to track, analyse and predict complaint patterns. over the next day, month and year.
- As a result, the transaction and customer data provided by a call centre was used effectively to reduce future complaints and generate business value
- Using Ayasdi, a major FS firm was able to achieve more than a 25% reduction in false positives and achieved savings of tens of millions of dollars - but there is still a lot more that can be done
DATA MONETISATION (Privitar)
- Privitar’s software solution allows the safe use of sensitive information enabling organisations to extract maximum data utility and economic benefit
- The sharp increase in data volume and usage in FS today has brought two competing dynamics: Data protection regulation aimed at protecting people from the misuse of their data and the absorption of data into tools/technologies such as machine learning
- However, as more data is made available, the harder it is to protect the privacy of the individual through data linkage
- Privitar’s tools are capable of removing a large amount of risk from this tricky area, and allow people to exchange data much more freely by anonymisation
- Privitar allows for open data for innovation and collaboration, whilst also acting in the best interest of customers’ privacy
SURVEY RESULTS
- Encouragingly, over 97% of participants who responded confirmed the five use cases presented were relevant to their respective organisations
- Nearly 50% of all participants who responded stated they would consider using the tech solutions presented
- 70% of responders believe their firms would be likely to adopt one of the solutions
- Only 10% of participants who responded believed the solutions were not relevant to their respective firms
- Approximately 30% of responders thought they would face difficulties in taking on a new solution