Strengthening Information Security
The Combined Power of Identity & Access Management and Data Access Controls
The digital age presents a double-edged sword for businesses. While technology advancements offer exciting capabilities in cloud, data analytics, and customer experience, they also introduce new security challenges. Data breaches are a constant threat, costing businesses an average of $4.45 million per incident according to a 2023 IBM report (https://www.ibm.com/reports/data-breach) and eroding consumer trust. Traditional security measures often fall short, leaving vulnerabilities for attackers to exploit. These attackers, targeting poorly managed identities and weak data protection, aim to disrupt operations, steal sensitive information, or even hold companies hostage. The impact extends beyond the business itself, damaging customers, stakeholders, and the broader financial market
In response to these evolving threats, the European Commission (EU) has implemented the Digital Operational Resilience Act (DORA) (Regulation (EU) 2022/2554). This regulation focuses on strengthening information and communications technology (ICT) resilience standards in the financial services sector. While designed for the EU, DORA’s requirements offer valuable insights for businesses globally, especially those with operations in the EU or the UK. DORA mandates that financial institutions define, approve, oversee, and be accountable for implementing a robust risk-management framework. This is where identity & access management (IAM) and data access controls (DAC).
The Threat Landscape and Importance of Data Security
Data breaches are just one piece of the security puzzle. Malicious entities also employ malware, phishing attacks, and even exploit human error to gain unauthorised access to sensitive data. Regulatory compliance further emphasises the importance of data security. Frameworks like GDPR and HIPAA mandate robust data protection measures. Failure to comply can result in hefty fines and reputational damage.
Organisations, in a rapidly-evolving hybrid working environment, urgently need to implement or review their information security strategy. This includes solutions that not only reduce the attack surface but also improve control over who accesses what data within the organisation. IAM and DAC, along with fine-grained access provisioning for various data formats, are critical components of a strong cybersecurity strategy.
Keep reading to learn the key differences between IAM and DAC, and how they work in tandem to create a strong security posture.
Identity & Access Management (IAM)
Think of IAM as the gatekeeper to your digital environment. It ensures only authorised users can access specific systems and resources. Here is a breakdown of its core components:
- Identity Management (authentication): This involves creating, managing, and authenticating user identities. IAM systems manage user provisioning (granting access), authentication (verifying user identity through methods like passwords or multi-factor authentication [MFA]), and authorisation (determining user permissions). Common identity management practices include:
- Single Sign-On (SSO): Users can access multiple applications with a single login, improving convenience and security.
- Multi-Factor Authentication (MFA):An extra layer of security requiring an additional verification factor beyond a password (e.g., fingerprint, security code).
- Passwordless: A recent usability improvement removes the use of passwords and replaces them with authentication apps and biometrics.
- Adaptive or Risk-based Authentication: Uses AI and machine learning to analyse user behaviour and adjust authentication requirements in real-time based on risk level.
- Access Management (authorisation): Once a user has had their identity authenticated, then access management checks to see what resources the user has access to. IAM systems apply tailored access policies based on user identities and other attributes. Once verified, IAM controls access to applications, data, and other resources.
Advanced IAM concepts like Privileged Access Management (PAM) focus on securing access for privileged users with high-level permissions, while Identity Governance ensures user access is reviewed and updated regularly.
Data Access Control (DAC)
While IAM focuses on user identities and overall system access, DAC takes a more granular approach, regulating access to specific data stored within those systems. Here are some common DAC models:
- Discretionary Access Control (also DAC): Allows data owners to manage access permissions for other users. While offering flexibility, it can lead to inconsistencies and security risks if not managed properly. One example of this is UNIX files, where an owner of a file can grant or deny other users access.
- Mandatory Access Control (MAC): Here, the system enforces access based on pre-defined security labels assigned to data and users. This offers stricter control but requires careful configuration.
- Role-Based Access Control (RBAC): This approach complements IAM RBAC by defining access permissions for specific data sets based on user roles.
- Attribute-Based Access Control (ABAC): Permissions are granted based on a combination of user attributes, data attributes, and environmental attributes, offering a more dynamic and contextual approach.
- Encryption: Data is rendered unreadable without the appropriate decryption key, adding another layer of protection.
IAM vs. DAC: Key Differences and Working Together
While IAM and DAC serve distinct purposes, they work in harmony to create a comprehensive security posture. Here is a table summarising the key differences:
FEATURE
IAM
DAC
Description
Controls access to applications
Controls access to data within applications
Granularity
Broader – manages access to entire systems
More fine-grained – controls access to specific data check user attributes
Enforcement
User-based (IAM) or system-based (MAC)
System-based enforcement (MAC) or user-based (DAC)
Imagine an employee accessing customer data in a CRM system. IAM verifies their identity and grants access to the CRM application. However, DAC determines what specific customer data they can view or modify based on their role (e.g., a sales representative might have access to contact information but not financial details).
Dispelling Common Myths
Several misconceptions surround IAM and DAC. Here is why they are not entirely accurate:
- Myth 1: IAM is all I need. The most common mistake that organisations make is to conflate IAM and DAC, or worse, assume that if they have IAM, that includes DAC. Here is a hint. It does not.
- Myth 2: IAM is only needed by large enterprises. Businesses of all sizes must use IAM to secure access to their applications and ensure compliance. Scalable IAM solutions are readily available.
- Myth 3: More IAM tools equal better security. A layered approach is crucial. Implementing too many overlapping IAM tools can create complexity and management overhead. Focus on choosing the right tools that complement each other and address specific security needs.
- Myth 4: Data access control is enough for complete security. While DAC plays a vital role, it is only one piece of the puzzle. Strong IAM practices ensure authorised users are accessing systems, while DAC manages their access to specific data within those systems. A comprehensive security strategy requires both.
Tools for Effective IAM and DAC
There are various IAM and DAC solutions available, and the best choice depends on your specific needs. While Active Directory remains a popular IAM solution for Windows-based environments, it may not be ideal for complex IT infrastructures or organisations managing vast numbers of users and data access needs.
Imagine a scenario where your application has 1,000 users and holds sensitive & personal customer information for 1,000,000 customers split across ten countries and five products. Not every user should see every customer record. It might be limited to the country the user works in and the specific product they support. This is the “Principle of Least Privilege.” Applying this principle is critical to demonstrating you have appropriate data access controls.
To control access to this data, you would need to create tens of thousands of AD groups for every combination of country or countries and product or products. This is unsustainable and makes choosing AD groups to manage data access control an extremely poor choice.
The complexity of managing nested AD groups and potential integration challenges with non-Windows systems highlight the importance of carefully evaluating your specific needs when choosing IAM tools. Consider exploring cloud-based IAM platforms or Identity Governance and Administration (IGA) solutions for centralised management and streamlined access control.
Building a Strong Security Strategy
The EU’s Digital Operational Resilience Act (DORA) emphasises strong IAM practices for financial institutions and will coming into act from 17 January 2025. DORA requires financial organisations to define, approve, oversee, and be accountable for implementing robust IAM and data access controls as part of their risk management framework.
Here are some key areas where IAM and DAC can help organisations comply with DORA and protect themselves:
DORA Pillar
How IAM helps
How DAC helps
ICT risk management
- Identifies risks associated with unauthorised access/misuse
- Detects users with excessive permissions or dormant accounts
- Minimises damage from breaches by restricting access to specific data
ICT related incident reporting
- Provides audit logs for investigating breaches (user activity, login attempts, accessed resources)
- Helps identify source of attack and compromised accounts
- Helps determine scope of breach and potentially affected information
ICT third-party risk management
- Manages access for third-party vendors/partners
- Grants temporary access with limited permissions, reducing attack surface
- Restricts access for third-party vendors by limiting ability to view/modify sensitive data
Information sharing
- Permissions designated users authorised to share sensitive information
- Controls access to shared information via roles and rules
Digital operational resilience testing
- Enables testing of IAM controls to identify vulnerabilities
- Penetration testing simulates attacks to assess effectiveness of IAM controls
- Ensures data access restrictions are properly enforced and minimizes breach impact
Understanding IAM and DAC empowers you to build a robust data security strategy
Use these strategies to leverage the benefits of IAM and DAC combined:
- Recognise the difference between IAM and DAC, and how they are implemented in your organisation
- Conduct regular IAM and DAC audits to identify and address vulnerabilities
- Implement best practices like the Principle of Least Privilege (granting users only the minimum access required for their job function)
- Regularly review and update user access permissions
- Educate employees on security best practices (e.g., password hygiene, phishing awareness)
Explore different IAM and DAC solutions based on your specific organisational needs and security posture. Remember, a layered approach that combines IAM, DAC, and other security measures like encryption creates the most effective defence against data breaches and unauthorised access.
Conclusion
By leveraging the combined power of IAM and DAC, you can ensure only the right people have access to the right data at the right time. This fosters trust with stakeholders, protects your reputation, and safeguards your valuable information assets.
Helping a leading insurance provider improve their data access controls
A global insurance provider had begun migrating their legacy on-premise applications to a new data lake. With a strategic reporting solution used, it was clear that report users had access to data that they did not need to have access to.
Previous studies had identified the gaps and it was time to push forward and deliver a solution. We were engaged to define the roles and data access control business rules to support Germany, as they had specific requirements around employee name visibility. A temporary solution had been implemented but a strategic solution that unmasked employee names to those who needed to see them, was required.
We developed the rules with support from the Claims business, the Data Protection Officer, and German Works Council. We designed and built a Power BI prototype to demonstrate the rules working using attribute-based access controls (ABAC).
This prototype and the business rules have led to a further engagement to implement the solution in a real report connected to the data lake.
Improving data access controls at a global insurer
"We approached Leading Point to support the enhancement of strategic data lake fine grained access controls capabilities. Their partnership approach working transversally across business and IT functions quickly surfaced root causes to be addressed as part of the improvement plan. Leading Point's approach to consulting services was particularly refreshing from a quality and cost stand point compared to some of the traditional players that we had consulted with before."
Head of Data Controls at Global Corporate Insurer
Helping a US broker-dealer manage its application estate using open source tools
Our client was a Fortune 500 US independent broker-dealer with over 17,500 financial advisors and over 1tn USD in advisory and brokerage assets. They had a large application estate with nearly 1,000 applications they had either developed, bought or acquired through mergers and takeovers. The applications were captured in ServiceNow CMDB but there was little knowledge around flows, owners, data, and batch jobs.
Additionally, the client also wanted to roll out a new data strategy. Part of this engagement with their business community was to educate and inform about the data strategy and its impact on their work.
We were asked to implement an open source enterprise architecture tool called Waltz. Waltz had been originally developed at Deutsche Bank and had recently been released as open source software under FINOS (Fintech Open Source Foundation). Waltz is not widely-known in financial services yet and we saw this as a great opportunity to demonstrate the benefits of using open source tools.
To support the data strategy rollout, the client asked if we could build a simple and clear internal website to show the new data strategy and data model. The data model would be navigable to drill-down into more detail and provide links to existing documentation.
Our approach:
With our extensive implementation experience, we put together a small, experienced, cross-border team to deploy and configure Waltz. We knew that understanding the client's data was key; what data was required, where was it, how good was its quality. Waltz uses data around:
- Organisational units - different structures depending on the viewpoint (business, technical)
- People - managerial hierarchies, roles, responsibilities
- Applications - owners, technologies, costs, licences, flows, batch jobs
- Data - hierarchies, entities, attributes, definitions, quality, owners, lineage
- Capabilities - owners, services, processes
- Change - initiatives, costs, impact
We split our work into a number of workstreams:
- Data readiness - understand what data they had, the sources, and the quality
- Data configuration - understand the relationships between the data and prepare it for Waltz
- Waltz implementation - understand the base open source version of Waltz with its limitations, gather the client requirements (like single-sign on and configurable data loaders), develop the features into Waltz, and deploy Waltz at the client
- Data strategy website - understand the audience, design website prototype options for client review, build an interactive React website for the rollout roadshows
The project was challenging because, as ever, the state of the data. There were multiple inconsistencies which hinders the use of tooling to bring order. We needed to identify those inconsistencies, see who should own them, and ensure they were resolved.
With the flexibility of an enterprise architecture tool, it was important to be clear around the specific problems we wanted to solve for the client. We identified 10+ potential use cases that we worked with the client to narrow down. Future extensions of the project enabled us to extend into these other use cases.
One such problem was around batch job documentation. The client had thousands of Word docs specifying batch jobs transferring data between internal and external applications. These documents were held in SharePoint, Confluence, and local drives. This made it difficult to find information about specific batch jobs if something went wrong, for example.
We used the applications captured in Waltz and linked them together. We developed a new data loader that could import Word docs and extract the batch job information automatically from them. This was used to populate Waltz and make this information searchable, reducing the time spent by Support teams to find out about failed jobs.
One common negative that is raised about similar applications is the effort involved to get data into the application. Waltz accelerates this by sending surveys out to crowd-source knowledge from across the organisation. We found this a great way of engaging with users and capturing their experience into Waltz.
Our results:
We were able to deploy an open source enterprise architecture tool on a client's AWS cloud within three months. This included adding new features, such as single sign-on, improving existing Waltz capabilities, like the data loaders, and defining the data standards to enable smooth data integrations with source systems.
Using Waltz showed the client the value of bringing together disparate knowledge from around the organisation into one place. It does expose data gaps, but we always see this as a benefit for the client, as any improvement in data quality yields improved business results.
Helping a UK retail bank to benchmark their ESG progress against their peers
Our client wanted to improve their ESG position against their competitors, based on real data. They were unsure about where to start with ESG measurement and integrating ESG philosophy into their culture and business processes.
We were asked to come up with an ESG scoring model that could use existing public data from the client's peers against their own internal reporting data. This scoring model would be used to place the client against their peers in environmental, social, and governance groups, as well as an overall rating. Our ESG expertise was recognised in identifying which ESG frameworks could support this scoring model. We were also tasked with ensuring that their ESG philosophy was aligned to their purpose.
Our approach:
We used an example of best-in-class ESG stewardship in a Tier 1 financial services firm as a demonstration of what is possible. This case study covered how ESG impacted the firm across:
- Partnerships
- Products & services
- Diversity & inclusion
- Climate change
- Governance & ESG frameworks
We created an ESG scoring model that used existing ESG frameworks, such as SASB and UN SDGs. This scoring model included 32 questions across E, S and G categories. We researched public company reports to find data and references to key ESG themes. Thresholds were used to classify metrics and create a weighted score per category.
We emphasised the importance of authenticity in embedding ESG into a firm's culture. This was demonstrated through analysis of peer behaviour and assessing ESG integration into the peers' purpose. A set of recommendations were made to increase the maturity of ESG within the client, including specific frameworks and metrics to start tracking.
Our results:
The board members at the client were able to see where they stood versus their competitors, in more detail than ever before. This detail enabled a set of specific next steps to be laid out around establishing the ESG philosophy and policy of the client, which ESG areas to prioritise, changes to the risk appetite statement to incorporate ESG risks, and making a commitment to becoming net-zero.
Helping Adjoint gain ISO 27001 information security certification to support its expansion strategy
Adjoint required ISO certification to comply with legislation, across multiple jurisdictions, and increase confidence in their brand. Due to the nature of their clients (fortune 500 and international companies), a widely recognised accreditation was required. The firm's incorporation of next generation processing, such as distributed ledger technology (DLT), increased the complexity to achieve certification. Their global teams in the UK, Switzerland and USA, were undergoing a heavy scaling-up.
We were asked to customise and implement an ISO 27001 framework for global accreditation in IT security management.
Our approach:
- Capture delivery requirements
- Create relevant policies, procedures and a controls framework, for applicable IT functions
- Perform gap analysis and risk assessment
- Establish clear roles and responsibilities and deliver a formal training program
- Conduct internal assurance audit to identify incidents and data breaches
- Lead external certification process with BSI, through Stage 1 and 2 completion
- Provide agile delivery through to completion
Our results:
- Effective coverage of all ISMS mandatory requirements surrounding ISO 27001
- A new performance management system to track controls in company processes, structure and focal points
- Global delivery, with clear road-mapping structure
- Scaled offerings in open APIs and raised brand in the market
- Improved sales process due to meeting client ISO requirements
Helping Clarivate Analytics define a financial services (FS) go-to-market strategy for intellectual property data
We were asked by Clarivate to analyse their IP data and identify where it might be useful in financial services, based on our industry experience. We created and reviewed 39 use cases, interviewed 59 financial services specialists, and reviewed 150 potential partner companies.
We developed four value propositions and recommended 16 projects to execute the strategy.
Helping a global investment bank design & execute a client data governance target operating model
Our client had a challenge to evidence control of their 2000+ client data elements. We were asked to implement a new target operating model for client data governance in six months. Our approach was to identify the core, essential data elements used by the most critical business processes and start governance for these, including data ownership and data quality.
We delivered business capability models, data governance processes, data quality rules & reporting, global support coverage for 100+ critical data elements supporting regulatory reporting and risk.
Helping a global investment bank reduce its residual risk with a target operating model
Our client asked us to provide operating model design & governance expertise for its anti-financial crime (AFC) controls. We reviewed and approved the bank’s AFC target operating model using our structured approach, ensuring designs were compliant with regulations, aligned to strategy, and delivered measurable outcomes.
We delivered clear designs with capability impact maps, process models, and system & data architecture diagrams, enabling change teams to execute the AFC strategy.
Helping Bloomberg improve its data offering for its customers
Bloomberg wanted us to help review and refresh their 80,000 data terms in order to build a clear ontology of related information. We identified & prioritised the core, essential terms and designed new business rules for the data relationships. By creating a system-based approach, we could train the Bloomberg team to continue our work as BAU.
We improved the definitions, domains, and ranges to align with new ontologies, enabling their 300,000 financial services professionals to make more informed investment decisions.
Helping GLEIF build out a new ISO standard for official organisational roles (ISO 5009)
GLEIF engaged us as financial services data experts to identify, analyse, and recommend relevant organisational roles for in-scope jurisdictions based on publicly-available laws & regulations. We looked at 12 locations in a four-week proof-of-concept, using automated document processing
Our work helped GLEIF to launch the ISO 5009 in 2022, enabling B2B verified digital signatures for individuals working in official roles. This digital verification speeds up onboarding time and increases trust.
Increasing data product offerings by profiling 80k terms at a global data provider
“Through domain & technical expertise Leading Point have been instrumental in the success of this project to analyse and remediate 80k industry terms. LP have developed a sustainable process, backed up by technical tools, allowing the client to continue making progress well into the future. I would have no hesitation recommending LP as a delivery partner to any firm who needs help untangling their data.”
PM at Global Market Data Provider
AI in Insurance - Article 1 - A Catalyst for Innovation
How insurance companies can use the latest AI developments to innovate their operations
The emergence of AI
The insurance industry is undergoing a profound transformation driven by the relentless advance of artificial intelligence (AI) and other disruptive technologies. A significant change in business thinking is gaining pace and Applied AI is being recognised for its potential in driving top-line growth and not merely a cost-cutting tool.
The adoption of AI is poised to reshape the insurance industry, enhancing operational efficiencies, improving decision-making, anticipating challenges, delivering innovative solutions, and transforming customer experiences.
This shift from data-driven to AI-driven operations is bringing about a paradigm shift in how insurance companies collect, analyse, and utilise data to make informed decisions and enhance customer experiences. By analysing vast amounts of data, including historical claims records, market forces, and external factors (global events like hurricanes, and regional conflicts), AI can assess risk with speed and accuracy to provide insurance companies a view of their state of play in the market.
Data vs AI approaches
This data-driven approach has enabled insurance companies to improve their underwriting accuracy, optimise pricing models, and tailor products to specific customer needs. However, the limitations of traditional data analytics methods have become increasingly apparent in recent years.
These methods often struggle to capture the complex relationships and hidden patterns within large datasets. They are also slow to adapt to rapidly-changing market conditions and emerging risks. As a result, insurance companies are increasingly turning to AI to unlock the full potential of their data and drive innovation across the industry.
AI algorithms, powered by machine learning and deep learning techniques, can process vast amounts of data far more efficiently and accurately than traditional methods. They can connect disparate datasets, identify subtle patterns, correlations & anomalies that would be difficult or impossible to detect with human analysis.
By leveraging AI, insurance companies can gain deeper insights into customer behaviour, risk factors, and market trends. This enables them to make more informed decisions about underwriting, pricing, product development, and customer service and gain a competitive edge in the ever-evolving marketplace.
Top 5 opportunities
1. Enhanced Risk Assessment
AI algorithms can analyse a broader range of data sources, including social media posts and weather patterns, to provide more accurate risk assessments. This can lead to better pricing and reduced losses.
2. Personalised Customer Experiences
AI can create personalised customer experiences, from tailored product recommendations to proactive risk mitigation guidance. This can boost customer satisfaction and loyalty.
3. Automated Claims Processing
AI can automate routine claims processing tasks, for example, by reviewing claims documentation and providing investigation recommendations, thus reducing manual efforts and improving efficiency. This can lead to faster claims settlements and lower operating costs.
4. Fraud Detection and Prevention
AI algorithms can identify anomalies and patterns in claims data to detect and prevent fraudulent activities. This can protect insurance companies from financial losses and reputational damage.
5. Predictive Analytics
AI can be used to anticipate future events, such as customer churn or potential fraud. This enables insurance companies to take proactive measures to prevent negative outcomes.
Adopting AI in Insurance
The adoption of AI in the insurance industry is not without its challenges. Insurance companies must address concerns about data quality, data privacy, transparency, and potential biases in AI algorithms. They must also ensure that AI is integrated seamlessly into their existing systems and processes.
Despite these challenges, AI presents immense opportunities. Insurance companies that embrace AI-driven operations will be well-positioned to gain a competitive edge, enhance customer experiences, and navigate the ever-changing risk landscape.
The shift from data-driven to AI-driven operations is a transformative force in the insurance industry. AI is not just a tool for analysing data; it is a catalyst for innovation and a driver of change. Insurance companies that harness the power of AI will be at the forefront of this transformation, shaping the future of insurance and delivering exceptional value to their customers.
Download the PDF article here.
Unlocking the opportunity of vLEIs
Streamlining financial services workflows with Verifiable Legal Entity Identifiers (vLEIs)
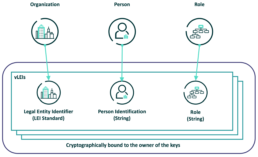
Source: GLIEF
Trust is hard to come by
How do you trust people you have never met in businesses you have never dealt with before? It was difficult 20 years ago and even more so today. Many checks are needed to verify if the person you are talking to is the person you think it is. Do they even work for the business they claim to represent? Failures of these checks manifest themselves every day with spear phishing incidents hitting the headlines, where an unsuspecting clerk is badgered into making a payment to a criminal’s account by a person claiming to be a senior manager.
With businesses increasing their cross-border business and more remote working, it is getting harder and harder to trust what you see in front of you. How do financial services firms reduce the risk of cybercrime attacks? At a corporate level, there are Legal Entity Identifiers (LEIs) which have been a requirement for regulated financial services businesses to operate in capital markets, OTC derivatives, fund administration or debt issuance.
LEIs are issued by Local Operating Units (LOUs). These are bodies that are accredited by GLEIF (Global Legal Entity Identifier Foundation) to issue LEIs. Examples of LOUs are the London Stock Exchange Group (LSEG) and Bloomberg. However, LEIs only work at a legal entity level for an organisation. LEIs are not used for individuals within organisations.
Establishing trust at this individual level is critical to reducing risk and establishing digital trust is key to streamlining workflows in financial services, like onboarding, trade finance, and anti-financial crime.
This is where Verifiable Legal Entity Identifiers (vLEIs) come into the picture.
What is the new vLEI initiative and how will it be used?
Put simply, vLEIs combine the organisation’s identity (the existing LEI), a person, and the role they play in the organisation into a cryptographically-signed package.
GLEIF has been working to create a fully digitised LEI service enabling instant and automated identity verification between counterparties across the globe. This drive for instant automation has been made possible by developments in blockchain technology, self-sovereign identity (SSI) and other decentralised key management platforms (Introducing the verifiable LEI (vLEI), GLEIF website).
vLEIs are secure digitally-signed credentials and a counterpart of the LEI, which is a unique 20-digit alphanumeric ISO-standardised code used to represent a single legal organisation. The vLEI cryptographically encompasses three key elements; the LEI code, the person identification string, and the role string, to form a digital credential of a vLEI. The GLEIF database and repository provides a breakdown of key information on each registered legal entity, from the registered location, the legal entity name, as well as any other key information pertaining to the registered entity or its subsidiaries, as GLEIF states this is of “principally ‘who is who’ and ‘who owns whom’”(GLEIF eBook: The vLEI: Introducing Digital I.D. for Legal Entities Everywhere, GLEIF Website).
In December 2022, GLEIF launched their first vLEI services through proof-of-concept (POC) trials, offering instant digitally verifiable credentials containing the LEI. This is to meet GLEIF’s goal to create a standardised, digitised service capable of enabling instant, automated trust between legal entities and their authorised representatives, and the counterparty legal entities and representatives with which they interact” (GLEIF eBook: The vLEI: Introducing Digital I.D. for Legal Entities Everywhere, page 2).
“The vLEI has the potential to become one of the most valuable digital credentials in the world because it is the hallmark of authenticity for a legal entity of any kind. The digital credentials created by GLEIF and documented in the vLEI Ecosystem Governance Framework can serve as a chain of trust for anyone needing to verify the legal identity of an organisation or a person officially acting on that organisation’s behalf. Using the vLEI, organisations can rely upon a digital trust infrastructure that can benefit every country, company, and consumers worldwide”,
Karla McKenna, Managing Director GLEIF Americas
This new approach for the automated verification of registered entities will benefit many organisations and businesses. It will enhance and speed up regulatory reports and filings, due diligence, e-signatures, client onboarding/KYC, business registration, as well as other wider business scenarios.
Imagine the spear phishing example in the introduction. A spoofed email will not have a valid vLEI cryptographic signature, so can be rejected (even automatically), saving potentially thousands of £.
How do I get a vLEI?
Registered financial entities can obtain a vLEI from a Qualified vLEI Issuer (QVI) organisation to benefit from instant verification, when dealing with other industries or businesses (Get a vLEI: List of Qualified vLEI Issuing Organisations, GLEIF Website).
A QVI organisation is authorised under GLEIF to register, renew or revoke vLEI credentials belonging to any financial entity. GLEIF offers a Qualification Program where organisations can apply to operate as a QVI. GLEIF maintain a list of QVIs on their website.

Source: GLIEF
What is the new ISO 5009:2022 and why is it relevant?
The International Organisation of Standards (ISO) published the ISO 5009 standard in 2022, which was initially proposed by GLEIF, for the financial services sector. This is a new scheme to address “the official organisation roles in a structured way in order to specify the roles of persons acting officially on behalf of an organisation or legal entity” (ISO 5009:2022, ISO.org).
Both ISO and GLEIF have created and developed this new scheme of combining organisation roles with the LEI, to enable digital identity management of credentials. This is because the ISO 5009 scheme offers a standard way to specify organisational roles in two types of LEI-based digital assets, being the public key certificates with embedded LEIs, as per X.509 (ISO/IEC 9594-8), also outlined in ISO 17442-2, or for digital verifiable credentials such as vLEIs to be specified, to help confirm the authenticity of a person’s role, who acts on behalf of an organisation (ISO 5009:2022, ISO Website). This will help speed up the validation of person(s) acting on behalf of an organisation, for regulatory requirements and reporting, as well as for ID verification, across various business use cases.
Leading Point have been supporting GLEIF in the analysis and implementation of the new ISO 5009 standard, for which GLEIF acts as the operating entity to maintain the ISO 5009 standard on behalf of ISO. Identifying and defining OORs was dependent on accurate assessments of hundreds of legal documents by Leading Point.
“We have seen first-hand the challenges of establishing identity in financial services and were proud to be asked to contribute to establishing a new standard aimed at solving this common problem. As data specialists, we continuously advocate the benefits of adopting standards. Fragmentation and trying to solve the same problem multiple times in different ways in the same organisation hurts the bottom line. Fundamentally, implementing vLEIs using ISO 5009 roles improves the customer experience, with quicker onboarding, reduced fraud risk, faster approvals, and most importantly, a higher level of trust in the business.”
Rajen Madan (Founder and CEO, Leading Point)
Thushan Kumaraswamy (Founding Partner & CTO, Leading Point)
How can Leading Point assist?
Our team of expert practitioners can assist financial entities to implement the ISO 5009 standard in their workflows for trade finance, anti-financial crime, KYC and regulatory reporting. We are fully-equipped to help any organisation that is looking to get vLEIs for their senior team and to incorporate vLEIs into their business processes, reducing costs, accelerating new business growth, and preventing anti-financial crime.
Glossary of Terms and Additional Information on GLEIF
Who is GLEIF?
The Global Legal Entity Identifier Foundation (GLEIF) was established by the Financial Stability Board (FSB) in June 2014 and as part of the G20 agenda to endorse a global LEI. The GLEIF organisation helps to implement the use of the Legal Entity Identifier (LEI) and is headquartered in Basel, Switzerland.
What is an LEI?
A Legal Entity Identifier (LEI) is a unique 20 alphanumeric character code based on the ISO-17442 standard. This is a unique identification code for legal financial entities that are involved in financial transactions. The role of the structure of how an LEI is concatenated, principally answers ‘who is who’ and ‘who owns whom’, as per ISO and GLEIF standards, for entity verification purposes and to improve data quality in financial regulatory reports.
How does GLEIF help?
GLEIF not only helps to implement the use of LEI, but it also offers a global reference data and central repository on LEI information via the Global LEI Index on gleif.org, which is an online, public, open, standardised, and a high-quality searchable tool for LEIs, which includes both historical and current LEI records.
What is GLEIF’S Vision?
GLEIF believe that each business involved in financial transactions should be identifiable with a unique single digital global identifier. GLEIF look to increase the rate of LEI adoption globally so that the Global LEI Index can include all global financial entities that engage in financial trading activities. GLEIF believes this will encourage market participants to reduce operational costs and burdens and will offer better insight into the global financial markets (Our Vision: One Global Identity Behind Every Business, GLEIF Website).
Séverine Raymond Soulier's Interview with Leading Point
Séverine Raymond Soulier’s Interview with Leading Point
Séverine Raymond Soulier is the recently appointed Head of EMEA at Symphony.com – the secure, cloud-based, communication and content sharing platform. Séverine has over a decade of experience within the Investment Banking sector and following 9 years with Thomson Reuters (now Refinitiv) where she was heading the Investment and Advisory division for EMEA leading a team of senior market development managers in charge of the Investing and Advisory revenue across the region. Séverine brings a wealth of experience and expertise to Leading Point, helping expand its product portfolio and its reach across international markets.
John Macpherson's Interview with Leading Point
John Macpherson’s Interview with Leading Point 2022
John Macpherson was the former CEO of BMLL Technologies; and is a veteran of the city, holding several MD roles at CITI, Nomura and Goldman Sachs. In recent years John has used his extensive expertise to advise start-ups and FinTech in challenges ranging from compliance to business growth strategy. John is Deputy Chair of the Investment Association Engine which is the trade body and industry voice for over 200+ UK investment managers and insurance companies.
Leading Point and P9 Form Collaboration to Accelerate Trade and Transaction Reporting
Leading Point and P9 Form Collaboration to Accelerate Trade and Transaction Reporting
Leading Point and Point Nine (P9) will collaborate to streamline and accelerate the delivery of trade and transaction reporting. Together, they will streamline the delivery of trade and transaction reporting using P9’s scalable regulatory solution, and Leading Point's data management expertise. This new collaboration will help both firms better serve their clients and provide faster, more efficient reporting.
London, UK, July 22nd, 2022
P9’s in-house proprietary technology is a scalable regulatory solution. It provides best-in-class reporting solutions to both buy- and sell-side financial firms, service providers, and corporations, such as ED&F Man, FxPro and Schnigge. P9 helps them ensure high-quality and accurate trade/transaction reporting, and to remain compliant under the following regimes: EMIR, MiFIR, SFTR, FinfraG, ASIC, CFTC and Canadian.
Leading Point, a highly regarded digital transformation company headquartered in London, are specialists in intelligent data solutions. They serve a global client base of capital market institutions, market data providers and technology vendors.
Leading Point are data specialists, who have helped some of the Financial Services industry’s biggest players organise and link their data, as well as design and deliver data-led transformations in global front-to-back trading. Leading Point are experts in getting into the detail of what data is critical to businesses. They deliver automation and re-engineered processes at scale, leveraging their significant financial services domain expertise.
The collaboration will combine the power of P9's knowledge of regulatory reporting, and Leading Point’s expertise in data management and data optimisation. The integration of Leading Point’s services and P9's regulatory technology will enable clients to seamlessly integrate improved regulatory reporting and efficient business processes.
Leading Point will organise and optimise P9’s client’s data sets, making it feasible for P9's regulatory software to integrate with client regulatory workflows and reporting. In a statement made by Christina Barbash, Business Development Manager at Point Nine, she claims that, “creating a network of best-in-breed partners will enable Point Nine to better serve its existing and potential clients in the trade and transaction reporting market.”
Andreas Roussos, Partner at Point Nine adds:
“Partnering with Leading Point is a pivotal strategic move for our organization. Engaging with consulting firms will not only give us a unique position in the market, but also allow us to provide more comprehensive service to our clients, making it a game-changer for our organization, our clients, and the industry as a whole.”
Dishang Patel, COO and Founding Partner at Leading Point, speaks on the collaboration:
“We are thrilled to announce that we are collaborating with Point Nine. Their technology and knowledge of regulatory reporting can assist the wider European market. The new collaboration will unlock doors to entirely new transformation possibilities for organisations within the Financial Sector across EMEA.”
The collaboration reflects the growing complexity of financial trading and businesses’ need for more automation for compliance with regulations, whilst ensuring data management is front and centre of the approach for optimum client success. Considering this, the two firms have declared to support organisations to improve the quality and accuracy of their regulatory reporting for all regimes.
About Leading Point
Leading Point is a digital transformation company with offices in London and Dubai. They are revolutionising the way change is done through their blend of expert services and their proprietary technology, modellr™.
Find out more at: www.leadingpoint.io
Contact Dishang Patel, Founding Partner & COO at Leading Point - dishang@leadingpoint.io
About Point Nine
Point Nine (Limassol, Cyprus), is a dedicated regulatory reporting firm, focusing on the provision of trade and transaction reporting services to legal entities across the globe. Point Nine uses its in-house cutting-edge proprietary technology to provide a best-in-class solution to all customers and regulatory reporting requirements.
Find out more at: www.p9dt.com
Contact Head office, Point Nine Data Trust Limited - info@p9dt.com
ESG Operating models hold the key to ESG compliance
John Macpherson on ESG Risk
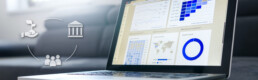
In my last article, I wrote about the need for an effective operating model in the handling and optimisation of data for Financial Services firms. But data is only one of several key trends amongst these firms that would benefit from a digital operating model. ESG has risen the ranks in importance, and the reporting of this has become imperative.
The Investment Association Engine Program, which I Chair, is designed to identify the most relevant pain points and key themes amongst Asset and Investment Management clients. We do this by searching out FinTech businesses that are already working on solutions to these issues. By partnering with these businesses, we can help our clients overcome their challenges and improve their operations.
While data has been an ever-present issue, ESG has risen to an equal standing of importance over the last couple of years. Different regulatory jurisdictions and expectations worldwide has left SME firms struggling to comply and implement in a new paradigm of environmental, sustainable and governance protocols.
ESG risk is different to anything we have experienced before and does not fit into neat categories such as areas like operational risk. The depth and breadth of data and models required for firms to make informed strategic decisions varies widely based on the specific issue at hand (e.g., supply chain, reputation, climate change goals, etc.). Firms need to carefully consider their own position and objectives when determining how much analysis is needed.
According to S&P Global, sustainable debt issuance reached a record level in 2021, and is only expected to increase further in the coming years. With this growth comes increased scrutiny and a heightened concern of so-called ‘greenwashing’, where companies falsely claim to be environmentally friendly. To combat this, participants need to manage that growth in a way that combats rising concerns about ‘greenwashing’.
Investors, regulators and the public, in general, are keen to challenge large companies’ ESG goals and results. These challenges vary wildly, but the biggest seen on a regular basis range from human rights to social unrest and climate change. As organisations begin to decarbonise their operations, they face the initially overlooked challenge of creating a credible near-term plan that will enable them to reach their long-term sustainability goals.
Investor pressure on climate change has historically focussed on the Energy sector. Now central banks are trying to incorporate climate risk as a stress testing feature for all Financial Services firms.
Source: S&P Global
Operating models hold the key to ESG transition and compliance. Having an operating model for how each of the firm’s functions intersect with ESG, requires new processes, new data, and new reporting techniques. This needs to be pulled across the enterprise, so firms have a process that is substantiated.
Before firms worry about ESG scores from their market data providers, they would do well to look closely at their own operating model and framework. In this way, they can then pull in the data required from the marketplace and use it in anger.
Leading Point is a FinTech business I am proud to be supporting. Their operating model system, modellr™ describes how financial services businesses work, from the products and services offered, to the key processes, people, data, and technology used to deliver value to their customers. This digital representation of how the business works is crucial to show what areas ESG will impact and how the firm can adapt in the most effective way.
Rajen Madan, CEO at Leading Point:
“In many ways, the transition to ESG is exposing the acute gap in firms of not being able to have meaningful dialogue with the plethora of data they already have, and need, to further add to for ESG”.
modellr™ harvests a company’s existing data to create a living dashboard, whilst also digitising the change process and enabling quicker and smarter decision-making. Access to all the information, from internal and external sources, in real time is proving transformative for SME size businesses.
Thushan Kumaraswamy, Chief Solutions Officer at Leading Point:
“ESG is already one of the biggest drivers of transformation in financial services and is only going to get bigger. Firms need to identify the impact on their business, choose the right change option, execute the strategy, and measure the improvements. The mass of ESG frameworks adds to the confusion of what to report and how. Tools such as modellr™ bring clarity and purpose to the ESG imperative.”
While most firms will look to sustainability officers for guidance on matters around ESG, Leading Point are providing these officers, and less qualified team members, with the tools to make informed decisions now, and in the future. We have established exactly what these firms need to succeed – a digital operating model.
Words by John Macpherson — Board advisor at Leading Point and Chair of the Investment Association Engine
The Challenges of Data Management
John Macpherson on The Challenges of Data Management
I often get asked, what are the biggest trends impacting the Financial Services industry? Through my position as Chair of the Investment Association Engine, I have unprecedented access to the key decision-makers in the industry, as well as constant connectivity with the ever-expanding Fintech ecosystem, which has helped me stay at the cutting edge of the latest trends.
So, when I get asked, ‘what is the biggest trend that financial services will face’, for the past few years my answer has remained the same, data.
During my time as CEO of BMLL, big data rose to prominence and developed into a multi-billion-dollar problem across financial services. I remember well an early morning interview I gave to CNBC around 5 years ago, where the facts were starkly presented. Back then, data was doubling every three years globally, but at an even faster pace in financial markets.
Firms are struggling under the weight of this data
The use of data is fundamental to a company's operations, but they are finding it difficult to get a handle on this problem. The pace of this increase has left many smaller and mid-sized IM/ AM firms in a quandary. Their ability to access, manage and use multiple data sources alongside their own data, market data, and any alternative data sources, is sub-optimal at best. Most core data systems are not architected to address the volume and pace of change required, with manual reviews and inputs creating unnecessary bottlenecks. These issues, among a host of others, mean risk management systems cannot cope as a result. Modernised data core systems are imperative to solve where real-time insights are currently lost, with fragmented and slow-moving information.
Around half of all financial service data goes unmentioned and ungoverned, this “dark data” poses a security and regulatory risk, as well as a huge opportunity.
While data analytics, big data, AI, and data science are historically the key sub-trends, these have been joined by data fabric (as an industry standard), analytical ops, data democratisation, and a shift from big data to smaller and wider data.
Operating models hold the key to data management
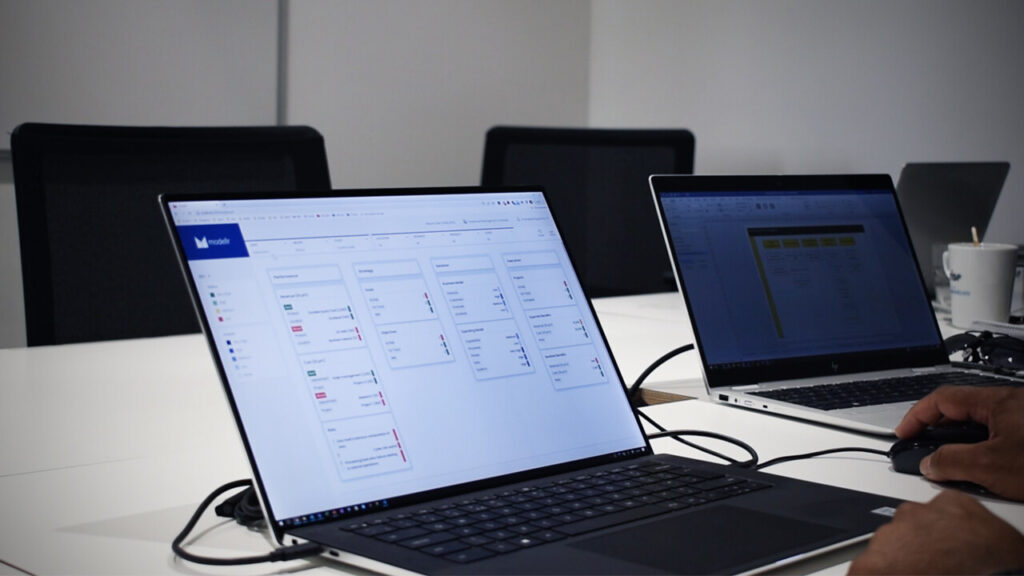
Governance is paramount to using this data in an effective, timely, accurate and meaningful way. Operating models are the true gauge as to whether you are succeeding.
Much can be achieved with the relatively modest budget and resources firms have, provided they invest in the best operating models around their data.
Leading Point is a firm I have been getting to know over several years now. Their data intelligence platform modellr™, is the first truly digital operating model. modellr™ harvests a company’s existing data to create a living operating model, digitising the change process, and enabling quicker, smarter, decision making. By digitising the process, they’re removing the historically slow and laborious consultative approach. Access to all the information in real-time is proving transformative for smaller and medium-sized businesses.
True transparency around your data, understanding it and its consumption, and then enabling data products to support internal and external use cases, is very much available.
Different firms are at very different places on their maturity curve. Longer-term investment in data architecture, be it data fabric or data mesh, will provide the technical backbone to harvest ML/ AI and analytics.
Taking control of your data
Recently I was talking to a large investment bank for whom Leading Point had been brought in to help. The bank was looking to transform its client data management and associated regulatory processes such as KYC, and Anti-financial crime.
They were investing heavily in sourcing, validating, normalising, remediating, and distributing over 2,000 data attributes. This was costing the bank a huge amount of time, money, and resources. But, despite the changes, their environment and change processes had become too complicated to have any chance of success. The process results were haphazard, with poor controls and no understanding of the results missing.
Leading Point was brought in to help and decided on a data minimisation approach. They profiled and analysed the data, despite working across regions and divisions. Quickly, 2,000 data attributes were narrowed to less than 200 critical ones for the consuming functions. This allowed the financial institutions, regulatory, and reporting processes to come to life, with clear data quality measurement and ownership processes. It allowed the financial institutions to significantly reduce the complexity of their data and its usability, meaning that multiple business owners were able to produce rapid and tangible results
I was speaking to Rajen Madan, the CEO of Leading Point, and we agreed that in a world of ever-growing data, data minimisation is often key to maximising success with data!
Elsewhere, Leading Point has seen benefits unlocked from unifying data models, and working on ontologies, standards, and taxonomies. Their platform, modellr™is enabling many firms to link their data, define common aggregations, and support knowledge graph initiatives allowing firms to deliver more timely, accurate and complete reporting, as well as insights on their business processes.
The need for agile, scalable, secure, and resilient tech infrastructure is more imperative than ever. Firms’ own legacy ways of handling this data are singularly the biggest barrier to their growth and technological innovation.
If you see a digital operating model as anything other than a must-have, then you are missing out. It’s time for a serious re-think.
Words by John Macpherson — Board advisor at Leading Point, Chair of the Investment Association Engine
John was recently interviewed about his role at Leading Point, and the key trends he sees affecting the financial services industry. Watch his interview here
Leading Point Shortlisted For Data Management Insight Awards
Leading Point has been shortlisted for the A-Teams Data Management Insight Awards.
Data Management Insight Awards, now in their seventh year, are designed to recognise leading providers of data management solutions, services and consultancy within capital markets.
Leading Point has been nominated for four categories:
- Most Innovative Data Management Provider
- Best Data Analytics Solution Provider
- Best Proposition for AI, Machine Learning, Data Science
- Best Consultancy in Data Management
Areas of Outstanding Service & Innovation
Leading Form Index: Data readiness assessment, created by Leading Point FM, which measures firms data capabilities and their capacity to transform across 24 unique areas. This allows participating firms to understand the maturity of their information assets, the potential to apply new tech (AI, DLT) and benchmark with peers.
Chief Risk Officer Dashboard: Management Information Dashboard that specifies, quantifies, and visualises risks arising from firms’ non-financial, operational, fraud, financial crime, and cyber risks.
Leading Point FM ‘Think Fast’ Application: The application provides the ability to input use cases and solution journeys and helps visualise process, systems and data flows, as well as target state definition & KPI’s. This allows business change and technology teams to quickly define and initiate change management.
Anti-Financial Crime Solution: Data centric approach combined with Artificial Intelligence technology reimagines and optimises AML processes to reduce volumes of client due diligence, reduce overall risk exposure, and provide the roadmap to AI-assisted automation.
Treasury Optimisation Solution: Data content expertise leveraging cutting edge DLT & Smart Contract technology to bridge intracompany data silos and enable global corporates to access liquidity and efficiently manage finance operations.
Digital Repapering Solution: Data centric approach to sourcing, management and distribution of unstructured data combined with NLP technology to provide roadmap towards AI assisted repapering and automated contract storage and distribution.
Leading Form Practical Business Design Canvas: A practical business design method to describe your business goals & objectives, change projects, capabilities, operating model, and KPI’s to enable a true business-on-a-page view that is captured within hours.
ISO 27001 Certification – Delivery of Information Security Management System (ISMS) & Cyber risk mitigation with a Risk Analysis Tool
GDFM & Leading Point Partnering for Smarter Regulatory Health Management
GDFM and Leading Point collaborate to deliver innovative and efficient regulatory risk management to our clients and through the SMART_Dash product; enabling consistent, centralised, accessible regulatory health data to assist responsible and accountable individuals with ensuring adequate transparency, for risk mitigation decision making and action taking. This is complemented by a SMART_Board suite for Board level leadership and a more detailed SMART_Support suite for regulatory reporting teams.
We are delighted that SMART_Dash has been shortlisted in 3 categories in this year's prestigious RegTech Insight Awards in Europe, which recognises both established solution providers and innovative newcomers, seeking to herald and highlight innovative RegTech solutions across the global financial services industry.
GD Financial Markets Head of Regulatory Compliance Practice and SMART_Dash Co-creator Sarah Peaston "Centralised, consolidated, consistent regulatory health transparency and tracking is key to identifying and managing regulatory and operating risk. I am delighted that SMART_Dash has been recognised as a new breed of solution that practically assists Managers, Senior Managers and Leadership with managing their regulatory health through the provision of the right information, at the right level to the right seniority”.
Leading Point CEO Rajen Madan "Our vision with SMART_Dash is to accelerate better regulatory risk management approaches and vastly more efficient RegOps. As financial services practitioners we are acutely aware of the time managers spend trying to make sense of their regulatory and operating risk areas from a multitude of inconsistent reports. SMART_Dash enables the shift to an enhanced way of risk management, which creates standardisation and makes reg data work for your business. We are very grateful to the COO, CRO and CFOs whom have contributed to its development and help the industry move forward”.
GDFM and Leading Point are rolling out the SMART_Dash suite to the first set of industry consortium partners progressively in H1 2021, and thereafter open to a wider set of institutions.
The Composable Enterprise: Improving the Front-Office User Experience
[et_pb_section fb_built="1" _builder_version="4.4.8" min_height="1084px" custom_margin="16px||-12px|||" custom_padding="0px||0px|||"][et_pb_row column_structure="2_3,1_3" _builder_version="3.25" custom_margin="-2px|auto||auto||" custom_padding="1px||3px|||"][et_pb_column type="2_3" _builder_version="3.25" custom_padding="|||" custom_padding__hover="|||"][et_pb_social_media_follow url_new_window="off" follow_button="on" _builder_version="4.4.8" text_orientation="left" module_alignment="left" min_height="14px" custom_margin="1px||5px|0px|false|false" custom_padding="0px|0px|0px|0px|false|false" border_radii="on|1px|1px|1px|1px"][et_pb_social_media_follow_network social_network="linkedin" url="https://uk.linkedin.com/company/leadingpoint" _builder_version="4.4.8" background_color="#007bb6" follow_button="on" url_new_window="off"]linkedin[/et_pb_social_media_follow_network][/et_pb_social_media_follow][et_pb_image src="https://leadingpointfm.com/wp-content/uploads/2020/10/cloud-based-services.png" title_text="cloud-based-services" align_tablet="center" align_phone="" align_last_edited="on|desktop" admin_label="Image" _builder_version="4.4.8" locked="off"][/et_pb_image][/et_pb_column][et_pb_column type="1_3" _builder_version="3.25" custom_padding="|||" custom_padding__hover="|||"][/et_pb_column][/et_pb_row][et_pb_row column_structure="1_2,1_2" _builder_version="4.4.8"][et_pb_column type="1_2" _builder_version="4.4.8"][et_pb_text _builder_version="4.4.8" text_font="||||||||" text_font_size="14px" text_line_height="1.6em" header_font="||||||||" header_font_size="25px" width="100%" custom_margin="10px|-34px|-5px|||" custom_padding="16px|0px|5px|8px||" content__hover_enabled="off|desktop"]
By Dishang Patel, Fintech & Growth Delivery Partner, Leading Point Financial Markets.
The past six months have by no means been a time of status quo. During this period of uncertainty, standards have been questioned and new ‘norms’ have been formed.
A standout development has been the intensified focus on cloud-based services. Levels of adoption have varied, from those moving to cloud for the first time, to others making cloud their only form of storage and access, and with numerous ‘others’ in between.
One area affected adversely (for those who weren’t ready) but positively (for those who were) is software. ‘Old-school’ software vendors – whose multi-million-pound solutions were traditionally implemented on premise at financial institutions, whether as part of a pure ‘buy’ or broader ‘build’ approach – have worked hard to offer cloud-based services.
The broad shift to working from home (WFH) as a result of the Covid-19 pandemic has tested the end-user experience all the way from front to back offices in financial institutions. Security, ease of access and speed are all high on the agenda in the new world in which we find ourselves.
The digitisation journey
With workforces operating globally, it is difficult to guarantee uniform user experiences and be able to cater for a multitude of needs. To achieve success in this area and to ensure a seamless WFH experience, financial institutions have moved things up a level and worked as hard as software providers to offer cloud-based solutions.
All manner of financial institutions (trading firms, brokerages, asset managers, challenger banks) have been on a digitisation journey to make the online user experience more consistent and reliable.
Composable Enterprise is an approach that those who have worked in a front office environment within financial services may have come across and for many could be the way forward.
Composable Enterprise: the way forward
Digitisation can come in many forms: from robotic process automation (RPA), operational excellence, implementation of application-based solution, interoperability and electronification. Interoperability and electronification are two key components of this Composable Enterprise approach.
Interoperability – whether in terms of web services, applications, or both – is an approach that can create efficiencies on the desktop and deliver improved user experience. It has the potential to deliver business performance benefits, in terms of faster and better decision making with the ultimate potential to uncover previously untapped alpha. It also has two important environmental benefits:
1) Reducing energy spend;
2) Less need for old hardware to be disposed of, delivering the reduced environmental footprint that organisations desire.
Electronification, for most industry players, may represent the final step on the full digitisation journey. According to the Oxford English Dictionary, electronification is the “conversion to or adoption of an electronic mode of operation,” which translates to the front office having all the tools they need to do their jobs to the best of their ability.
The beauty of both interoperability and electronification is that they work just as well in a remote set up as they do in an office environment. This is because a good implementation of both results in maximising an organisation’s ability to use all the tools (trading platforms, market data feeds, CRMs, and so on) at their disposal without needing masses of physical infrastructure.
Because of the lower barriers (such as time and cost) of interoperability, financial institutions should start their digitisation journeys from this component and then embark on a larger and more complicated move to electronification.
Composable Enterprise is about firms being able to choose the best component needed for their business, allowing them to be more flexible and more open in order to adapt to new potential revenue opportunities. In these challenging times, it is no surprise that more and more financial institutions are adding Composable Enterprise as a key item on their spending agenda.
[/et_pb_text][/et_pb_column][et_pb_column type="1_2" _builder_version="4.4.8"][et_pb_text disabled_on="on|on|off" _builder_version="4.4.8" min_height="15px" custom_margin="452px||133px|||" custom_padding="8px||0px|||"]
"The broad shift to working from home as a result of the Covid-19 pandemic has tested the end-user experience all the way from front to back offices in financial institutions."
[/et_pb_text][et_pb_text disabled_on="on|on|off" _builder_version="4.4.8" min_height="15px" custom_margin="452px||133px|||" custom_padding="8px|||||"]
"It has the potential to deliver business performance benefits, in terms of faster and better decision making with the ultimate potential to uncover previously untapped alpha."
[/et_pb_text][et_pb_text disabled_on="on|on|off" _builder_version="4.4.8" min_height="15px" custom_margin="427px|||||" custom_padding="1px|||||"]
"The beauty of both interoperability and electronification is that they work just as well in a remote set up as they do in an office environment."
[/et_pb_text][/et_pb_column][/et_pb_row][/et_pb_section][et_pb_section fb_built="1" _builder_version="3.22.3" animation_style="fade" locked="off"][et_pb_row _builder_version="3.25"][et_pb_column type="4_4" _builder_version="3.25" custom_padding="|||" custom_padding__hover="|||"][et_pb_team_member name="Dishang Patel" position="Fintech & Growth Delivery Partner" image_url="https://leadingpointfm.com/wp-content/uploads/2020/03/dishang.2e16d0ba.fill-400x400-1.jpg" _builder_version="4.4.8" link_option_url="mailto:dishang@leadingpoint.io" hover_enabled="0" admin_label="Person" title_text="dishang.2e16d0ba.fill-400x400"]
Responsible for delivering digital FS businesses.
Transforming delivery models for the scale up market.
[/et_pb_team_member][et_pb_text admin_label="Contact Us" module_class="txtblue" _builder_version="3.27.4" text_font="||||||||" link_font="||||||||" ul_font="||||||||" text_orientation="center"]
Contact Us
[/et_pb_text][et_pb_text admin_label="Form" _builder_version="3.27.4"][formidable id=2][/et_pb_text][et_pb_code admin_label="Social media icons" module_class="form" _builder_version="3.19.4" custom_margin="0px||0px" custom_padding="0px||0px"]
-
- Connect
[/et_pb_code][/et_pb_column][/et_pb_row][/et_pb_section]
Information Security in a New Digital Era
[et_pb_section fb_built="1" _builder_version="4.4.8" min_height="1084px" custom_margin="16px||-12px|||" custom_padding="0px||0px|||"][et_pb_row column_structure="2_3,1_3" _builder_version="3.25" custom_margin="-2px|auto||auto||" custom_padding="1px||3px|||"][et_pb_column type="2_3" _builder_version="3.25" custom_padding="|||" custom_padding__hover="|||"][et_pb_social_media_follow url_new_window="off" follow_button="on" admin_label="Social Media Follow" _builder_version="4.4.8" text_orientation="left" module_alignment="left" min_height="14px" custom_margin="1px||5px|0px|false|false" custom_padding="0px|0px|0px|0px|false|false" border_radii="on|1px|1px|1px|1px"][et_pb_social_media_follow_network social_network="linkedin" url="https://uk.linkedin.com/company/leadingpoint" _builder_version="4.4.8" background_color="#007bb6" follow_button="on" url_new_window="off"]linkedin[/et_pb_social_media_follow_network][/et_pb_social_media_follow][et_pb_image src="https://leadingpointfm.com/wp-content/uploads/2020/09/infosec.jpg" title_text="infosec" align_tablet="center" align_phone="" align_last_edited="on|desktop" admin_label="Image" _builder_version="4.4.8" locked="off"][/et_pb_image][/et_pb_column][et_pb_column type="1_3" _builder_version="3.25" custom_padding="|||" custom_padding__hover="|||"][/et_pb_column][/et_pb_row][et_pb_row column_structure="1_2,1_2" _builder_version="4.4.8"][et_pb_column type="1_2" _builder_version="4.4.8"][et_pb_text admin_label="Text" _builder_version="4.4.8" text_font="||||||||" text_font_size="14px" text_line_height="1.6em" header_font="||||||||" header_font_size="25px" width="100%" custom_margin="10px|-34px|-5px|||" custom_padding="16px|0px|5px|8px||" content__hover_enabled="off|desktop"]
Shifting priorities
The 2020’s pandemic, subsequent economic turmoil and related social phenomena has paved the way for much-needed global digital transformation and the prioritisation of digital strategies. The rise in digitisation across all businesses, however, has accelerated cyber risk exponentially. With cloud-based attacks rising by 630% between January and April 2020(1), organisations are now turning their focus on how to benefit from digitisation whilst maintaining sufficiently secure digital environments for their services and clients.
A global challenge
A new digital setup could easily jeopardise organisations’ cyber safety. With data becoming companies’ most valuable asset, hackers are getting creative with increasingly-sophisticated threats and phishing attacks. According to the 2019 Data Breach Investigation Report(2) by Verizon, 32% of all verified data breaches appeared to be phishing.
As data leaks are increasing (3,800 alone in 2019), so is the cyber skill shortage. According to the MIT Technology Review report(3), there will be 3.5 million unfulfilled cybersecurity jobs in 2021; a rise of 350%. As a result of Covid-19 and digitised home working, cybersecurity professionals are high in demand to fill the gaps organisations’
currently face.
The way forward
Although tackling InfoSec breaches in the rapidly-evolving digital innovation landscape is not easy, it is essential to keep it as an absolute priority. In our work with regulated sector firms in financial services, pharma and energy as well as with fintechs, we see consistent steps that underpin successful information security risk management. We have created a leaderboard of 10 discussion points for COOs, CIOs and CISOs to keep up with their information security needs:
- Information Security Standards
Understand information security standards like NIST, ISO 27001/2 and BIP 0116/7 and put in place processes and controls accordingly. These are good practices to keep a secure digital environment and are vital to include in your risk mitigation strategy. Preventing cyber attacks and data breaches is less costly and less resource-exhaustive than dealing with the damage caused by these attacks. There are serious repercussions of security breaches in terms of cost and reputational damage, yet organisations still only look at the issue after the event. Data shows that firms prefer to take a passive approach to tackle these issues instead of taking steps to prevent them in the first place.
- Managing security in cloud delivery models
2020 has seen a rise in the use of SaaS applications to support employee engagement, workflow management and communication. While cloud is still an area in its preliminary stages, cloud adoption is rapidly accelerating. But many firms have initiated cloud migration projects without a firm understanding and design for the future business, customer or end user flows. This is critical to ensuring a good security infrastructure in a multi-cloud operating environment. How does your firm keep up with the latest developments in Cloud Management?
- Operational resilience
70% of Operational Risk professionals say that their priorities and focus have changed as a result of Covid-19(4). With less than half of businesses testing their continuity and business-preparedness initiatives(5), Coronavirus served as an eye-opener in terms of revisiting these questions. Did your business continuity plan prove successful? If so, what was the key to its success? How do you define and measure operational resilience in your business? Cross-functional data sets are increasingly vital for informed risk management.
- Culture
Cyber risk is not just a technology problem; it is a people
problem. You cannot mitigate cyber risks with just technology;
embedding the right culture within your team is vital. How do you make sure a cyber-secure company culture is kept up in remote working environments? Does your company already have an information security training plan in place?
- Knowing what data is important
Data is expanding exponentially – you have to know what you need to protect. Only by defining important data, reducing the signal-to-data noise and aggregating multiple data points can organisations look to protect them. As a firm, what percentage of your data elements are defined with an owner and user access workflow?
- Speed of innovation means risk
The speed of innovation is often faster than the speed of safety. As technology and data adoption is rapidly changing, data protection has to keep up as well – there is little point in investing in technology until you really understand your risks and your exposure to those risks. This is increasingly true of new business-tech frameworks, including DLT, AI and Open Banking. When looking at DLT and AI based processes - how do you define the security and thresholds?
- Master the basics
80% of UK companies and startups are not Cyber Essentials ready, which shows that the fundamentals of data security are not being dealt with. Larger companies are rigid and not sufficiently agile – more demands are being placed on teams but without sufficient resources and skills development. Large companies cannot innovate if they are not given the freedom to actually adapt. What is the blocker in your firm?
- Collaborate with startups
Thousands of innovative startups tackling cyber security currently exist and many more will begin their growth journey over the next few years. Larger businesses need to be more open to collaborating with them to help speed up advancements in the cyber risk space.
- The right technology can play a key role in efficiency and speed
We see the emerging operating models for firms are open API based, and organisations need to stitch together many point solutions. Technology can help here if deployed correctly. For
instance, to join up multiple data, to provide transparency of
messages crossing in and out of systems, to execute and detect
information security processes and controls with 100x efficiency and speed. This will make a material difference in the new world of
financial services.
- Transparency of your supply chain
Supply chains are becoming more data-driven than ever with increased number of core operations and IT services being outsourced. Attackers are using weak supplier controls to compromise client networks and dispersed dependencies create increased reliance and risk exposure from entities outside of your direct control. How do you manage the current pressure points of your supplier relationships?
Next steps
Cyber risk (especially regarding data protection) is simultaneously a compliance problem (regulatory risk, legal risk etc.), an architecture problem (infrastructure, business continuity, etc.), and a business problem (reputational risk, loss of trust, ‘data poisoning’, competitor intelligence etc.). There are existing risk assessment frameworks for managing operational risk (example: ORMF) – why not plug in?
Getting the basics right, using industry standards, multi-cloud environments and transparency of supply chain are good places to start. These are all to do with holistic data risk management (HRM).
While all these individual issues pose problems on their own, they can be viewed through inter-relationships applying a holistic approach where a coordinated solution can be found to efficiently manage these issues as a whole. The solution lies in taking a more deliberate approach to cyber security and following this 4-step process:
IDENTIFY
ORGANISE
ASSIGN
RESOLVE
Find out more on Operational Resilience from Leading Point:
https://leadingpointfm.com/operational-resilience-data-infrastructure-and-aconsolidated-risk-view-is-pivotal-to-the-new-rules-on-operational-risk/#_edn2
Find out more on Data Kitchen, a Leading Point initiative:
https://leadingpointfm.com/the-data-kitchen-does-data-need-science/
(1) https://www.fintechnews.org/the-2020-cybersecurity-stats-you-need-to-know/
(2) https://www.techfunnel.com/information-technology/cyber-security-trends/
(5) https://securityintelligence.com/articles/these-cybersecurity-trends-could-get-a-boost-in-2020/
[/et_pb_text][/et_pb_column][et_pb_column type="1_2" _builder_version="4.4.8"][et_pb_text disabled_on="on|on|off" _builder_version="4.4.8" min_height="15px" custom_margin="452px||133px|||" custom_padding="8px|||||"]
"With data becoming companies’ most valuable asset, hackers are getting creative with increasingly-sophisticated threats and phishing attacks."
[/et_pb_text][et_pb_text disabled_on="on|on|off" _builder_version="4.4.8" min_height="15px" custom_margin="452px||133px|||" custom_padding="8px||0px|||"]
"Preventing cyber attacks and data breaches is less costly and less resource-exhaustive than dealing with the damage caused by these attacks."
[/et_pb_text][et_pb_text disabled_on="on|on|off" _builder_version="4.4.8" min_height="15px" custom_margin="427px|||||" custom_padding="1px|||||"]
"70% of Operational Risk professionals say that their priorities and focus have changed as a result of Covid-19."
[/et_pb_text][/et_pb_column][/et_pb_row][/et_pb_section][et_pb_section fb_built="1" _builder_version="4.4.8"][et_pb_row column_structure="1_3,1_3,1_3" _builder_version="4.4.8" min_height="643px"][et_pb_column type="1_3" _builder_version="4.4.8"][et_pb_gallery gallery_ids="4011" show_title_and_caption="off" _builder_version="4.4.8" width="100%"][/et_pb_gallery][et_pb_text _builder_version="4.4.8" custom_margin="-82px|||||" custom_padding="0px|||||"]
Rajen Madan
Founder & CEO
rajen@leadingpoint.io
Delivering Digital FS businesses. Change leader with over 20 years’ experience in helping firms with efficiency, revenue and risk management challenges
[/et_pb_text][/et_pb_column][et_pb_column type="1_3" _builder_version="4.4.8"][et_pb_image src="https://leadingpointfm.com/wp-content/uploads/2020/09/Aliz-photo-colour-320x500-1.jpg" title_text="Aliz photo colour 320x500 (1)" _builder_version="4.4.8"][/et_pb_image][et_pb_text _builder_version="4.4.8"]
Aliz Gyenes
Leading Point
Data Innovation, InfoSec, Investment behaviour research Helping businesses understand and improve their data strategy via the Leading Point Data Innovation Index
[/et_pb_text][/et_pb_column][et_pb_column type="1_3" _builder_version="4.4.8"][/et_pb_column][/et_pb_row][/et_pb_section][et_pb_section fb_built="1" module_class="txtwhite" _builder_version="3.22.3" background_color="#23408f" custom_padding="||62px|||" locked="off"][et_pb_row _builder_version="4.4.8"][et_pb_column type="4_4" _builder_version="4.4.8"][et_pb_text _builder_version="4.4.8" text_text_color="#ffffff" text_font_size="15px" header_text_color="#ffffff"]
How Leading Point can help
[/et_pb_text][/et_pb_column][/et_pb_row][et_pb_row column_structure="1_3,1_3,1_3" _builder_version="4.4.8"][et_pb_column type="1_3" _builder_version="4.4.8"][/et_pb_column][et_pb_column type="1_3" _builder_version="4.4.8"][/et_pb_column][et_pb_column type="1_3" _builder_version="4.4.8"][/et_pb_column][/et_pb_row][/et_pb_section][et_pb_section fb_built="1" _builder_version="4.4.8" animation_style="fade" locked="off"][et_pb_row _builder_version="3.25"][et_pb_column type="4_4" _builder_version="3.25" custom_padding="|||" custom_padding__hover="|||"][et_pb_text admin_label="Contact Us" module_class="txtblue" _builder_version="3.27.4" text_font="||||||||" link_font="||||||||" ul_font="||||||||" text_orientation="center"]
Contact Us
[/et_pb_text][et_pb_text admin_label="Form" _builder_version="3.27.4"][formidable id=2][/et_pb_text][et_pb_code admin_label="Social media icons" module_class="form" _builder_version="3.19.4" custom_margin="0px||0px" custom_padding="0px||0px"]
-
- Connect
[/et_pb_code][/et_pb_column][/et_pb_row][/et_pb_section]
Artificial Intelligence: The Solution to the ESG Data Gap?
The Power of ESG Data
It was Warren Buffett who said, “It takes twenty years to build a reputation and five minutes to ruin it” and that is the reality that all companies face on a daily basis. An effective set of ESG (Environment, Social & Governance) policies has never been more crucial. However, it is being hindered by difficulties surrounding the effective collection and communication of ESG data points, as well a lack of standardisation when it comes to reporting such data. As a result, the ESG space is being revolutionised by Artificial Intelligence, which can find, analyse and summarise this information.
There is increasing public and regulatory pressure on firms to ensure their policies are sustainable and on investors to take such policies into account when making investment decisions. The issue for investors is how to know which firms are good ESG performers and which are not. The majority of information dominating research and ESG indices comes from company-reported data. However, with little regulation surrounding this, responsible investors are plagued by unhelpful data gaps and “Greenwashing”. This is when a firm uses favourable data points and convoluted wording to appear more sustainable than they are in reality. They may even leave out data points that reflect badly on them. For example, firms such as Shell are accused of using the word ‘sustainable’ in their mission statement whilst providing little evidence to support their claims (1).
Could AI be the complete solution?
AI could be the key to help investors analyse the mountain of ESG data that is yet to be explored, both structured and unstructured. Historically, AI has been proven to successfully extract relevant information from data sources including news articles but it also offers new and exciting opportunities. Consider the transcripts of board meetings from a Korean firm: AI could be used to translate and examine such data using techniques such as Sentiment Analysis. Does the CEO seem passionate about ESG issues within the company? Are they worried about an investigation into Human Rights being undertaken against them? This is a task that would be labour-intensive, to say the least, for analysts to complete manually.
In addition, AI offers an opportunity for investors to not only act responsibly, but also align their ESG goals to a profitable agenda. For example, algorithms are being developed that can connect specific ESG indicators to financial performance and can therefore be used by firms to identify the risk and reward of certain investments.
Whilst AI offers numerous opportunities with regards to ESG investing, it is not without fault. Firstly, AI takes enormous amounts of computing power and, hence, energy. For example, in 2018, OpenAI found the level of computational power used to train the largest AI models has been doubling every 3.4 months since 2012 (2). With the majority of the world’s energy coming from non-renewable sources, it is not difficult to spot the contradiction in motives here. We must also consider whether AI is being used to its full potential; when simply used to scan company published data, AI could actually reinforce issues such as “Greenwashing”. Further, the issue of fake news and unreliable sources of information still plagues such methods and a lot of work has to go into ensuring these sources do not feature in algorithms used.
When speaking with Dr Thomas Kuh, Head of Index at leading ESG data and AI firm Truvalue Labs™, he outlined the difficulties surrounding AI but noted that since it enables human beings to make more intelligent decisions, it is surely worth having in the investment process. In fact, he described the application of AI to ESG research as ‘inevitable’ as long as it is used effectively to overcome the shortcomings of current research methods. For instance, he emphasised that AI offers real time information that traditional sources simply cannot compete with.
A Future for AI?
According to a 2018 survey from Greenwich Associates (3), only 17% of investment professionals currently use AI as part of their process; however, 40% of respondents stated they would increase budgets for AI in the future. As an area where investors are seemingly unsatisfied with traditional data sources, ESG is likely to see more than its fair share of this increase. Firms such as BNP Paribas (4) and Ecofi Investissements (5) are already exploring AI opportunities and many firms are following suit. We at Leading Point see AI inevitably becoming integral to an effective responsible investment process and intend to be at the heart of this revolution.
AI is by no means the judge, jury and executioner when it comes to ESG investing and depends on those behind it, constantly working to improve the algorithms, as well as the analysts using it to make more informed decisions. AI does, however, have the potential to revolutionise what a responsible investment means and help reallocate resources towards firms that will create a better future.
[1] The problem with corporate greenwashing
[2] AI and Compute
[3] Could AI Displace Investment Bank Research?
[4] How AI could shape the future of investment banking
[5] How AI Can Help Find ESG Opportunities
"It takes twenty years to build a reputation and five minutes to ruin it"
AI offers an opportunity for investors to not only act responsibly, but also align their ESG goals to a profitable agenda
Environmental Social Governance (ESG) & Sustainable Investment
Client propositions and products in data driven transformation in ESG and Sustainable Investing. Previous roles include J.P. Morgan, Morgan Stanley, and EY.
Upcoming blogs:
This is the second in a series of blogs that will explore the ESG world: its growth, its potential opportunities and the constraints that are holding it back. We will explore the increasing importance of ESG and how it affects business leaders, investors, asset managers, regulatory actors and more.
Riding the ESG Regulatory Wave: In the third part of our Environmental, Social and Governance (ESG) blog series, Alejandra explores the implementation challenges of ESG regulations hitting EU Asset Managers and Financial Institutions.
Is it time for VCs to take ESG seriously? In the fourth part of our Environmental, Social and Governance (ESG) blog series, Ben explores the current research on why startups should start implementing and communicating ESG policies at the core of their business.
Now more than ever, businesses are understanding the importance of having well-governed and socially-responsible practices in place. A clear understanding of your ESG metrics is pivotal in order to communicate your ESG strengths to investors, clients and potential employees.
By using our cloud-based data visualisation platform to bring together relevant metrics, we help organisations gain a standardised view and improve your ESG reporting and portfolio performance. Our live ESG dashboard can be used to scenario plan, map out ESG strategy and tell the ESG story to stakeholders.
AI helps with the process of ingesting, analysing and distributing data as well as offering predictive abilities and assessing trends in the ESG space. Leading Point is helping our AI startup partnerships adapt their technology to pursue this new opportunity, implementing these solutions into investment firms and supporting them with the use of the technology and data management.
We offer a specialised and personalised service based on firms’ ESG priorities. We harness the power of technology and AI to bridge the ESG data gap, avoiding ‘greenwashing’ data trends and providing a complete solution for organisations.
Leading Point's AI-implemented solutions decrease the time and effort needed to monitor current/past scandals of potential investments. Clients can see the benefits of increased output, improved KPIs and production of enhanced data outputs.
Implementing ESG regulations and providing operational support to improve ESG metrics for banks and other financial institutions. Ensuring compliance by benchmarking and disclosing ESG information, in-depth data collection to satisfy corporate reporting requirements, conducting appropriate investment and risk management decisions, and to make disclosures to clients and fund investors.
ESG: The Future Pillars of Investing
The ESG Explosion
With the ESG (Environmental, Social and Governance) market being estimated to reach $50 trillion over the next two decades [i], it is safe to say ESG is here to stay. This explosion is being driven by an increasingly conscientious world, with voices such as Greta Thunberg ensuring we no longer stay passive in our impact. Investors are increasingly realising the gains to be had from aligning themselves with firms that perform well in ESG criteria, such as risk management and possible financial gains.
This movement from investors as well as the general public has motivated firms to look in the mirror with regards to ESG performance and how they can improve. With new regulation on the horizon, forward thinking companies are wanting to report their ESG data more frequently and comprehensively.
ESG is Good for Business
ESG investing is becoming increasingly driven by millennials, who are taking an active role in aligning their personal values and their investing strategies. This investment pattern facilitates the belief that change - now more than ever - is a goal we can reach. If consumer behaviour is more directed towards ‘creating an impact’, what is the logical next step for businesses to thrive?
Organisations need to become more conscious of their mission and how they communicate it to the public, especially since good ESG metrics and reporting could seriously affect their staff and customer base[ii]
. Today’s start-up culture and the focus on the entrepreneurial mindset further demands this issue to be taken seriously. As well as helping to land conscientious clients and retain millennial job talent, a strong ESG proposition directly correlates to value creation within a business. More than a fad or a feel good exercise,[iii] a stronger esg proposition correlates with higher equity returns.
Why ESG is Important for Investors
During Q2 2019, ETFs with a sustainability criteria attracted EUR5 billion in net flows; this is more than throughout the whole of 2018[iv]. As demand skyrockets for responsible funds, there is increasing client pressure on investors and asset managers to take ESG factors into consideration. However, there are many other reasons why ESG data provides a competitive edge to investors.
Firstly, a good ESG performance is a strong indicator that a business is well-managed and, hence, considering ESG data acts as an effective way to manage risk. For example, a recent report from McKinsey states good ESG performance is associated with lower loan and credit default swap spreads and higher credit ratings.[v]
As well as a desire to profit from ESG data, there is ever-tightening regulation meaning investors need to care about it. For example, the EU taxonomy regulation is redefining what it means for an investment to be ‘environmentally sustainable’. Investors are keen to stay ahead of such regulation by having effective methods to monitor the key ESG data points of their portfolio companies.
Constraints on ESG
Whilst the ESG market is growing incredibly fast, there are a number of constraints on this growth. Financial data has clear, widely-agreed metrics whose implications are straightforward; however, the same cannot be said for ESG data. This can result in an “ESG Data Gap” between businesses and their investors as ESG information is failed to be communicated effectively between the two parties.
This “Data Gap” is especially obvious in the startup world where sustainable VCs are failing to communicate the ESG landscape of their portfolio companies effectively to their LPs. Finally, there is also ever-tightening regulation surrounding ESG disclosure for Asset Managers and FIs generally. It is difficult to integrate these effectively into procedures leading to inefficiencies.
Our series of blogs will delve deeper into the ESG world and these problems which plague it.
[i] Complete guide to sustainable investing
[ii] Five ways that ESG creates value
[iv] https://www.wealthadviser.co/2020/01/06/281642/how-artificial-intelligence-transforming-esg-data-and-indices
[iv] https://www.mckinsey.com/business-functions/strategy-and-corporate-finance/our-insights/five-ways-that-esg-creates-value
As demand skyrockets for responsible funds, there is increasing client pressure on investors and asset managers to take ESG factors into consideration.
a good ESG performance is a strong indicator that a business is well-managed and, hence, considering ESG data acts as an effective way to manage risk.
Data Innovation, Investment behaviour research
Environmental Social Governance (ESG) & Sustainable Investment
Client propositions and products in data driven transformation in ESG and Sustainable Investing. Previous roles include J.P. Morgan, Morgan Stanley, and EY.
Upcoming blogs:
This is the first in a series of blogs that will explore the ESG world: its growth, its potential opportunities and the constraints that are holding it back. We will explore the increasing importance of ESG and how it affects business leaders, investors, asset managers, regulatory actors and more.
Artificial Intelligence: the Solution to the ESG Data Gap? In the second part of our Environmental, Social and Governance (ESG) blog series, Anya explores the potential opportunities surrounding Artificial Intelligence and responsible investing.
Riding the ESG Regulatory Wave: In the third part of our Environmental, Social and Governance (ESG) blog series, Alejandra explores the implementation challenges of ESG regulations hitting EU Asset Managers and Financial Institutions.
Is it time for VCs to take ESG seriously? In the fourth part of our Environmental, Social and Governance (ESG) blog series, Ben explores the current research on why startups should start implementing and communicating ESG policies into their business.
Now more than ever, businesses are understanding the importance of having well-governed and socially-responsible practices in place. A clear understanding of your ESG metrics is pivotal in order to communicate your ESG strengths to investors, clients and potential employees.
By using our cloud-based data visualisation platform to bring together relevant metrics, we help organisations gain a standardised view and improve your ESG reporting and portfolio performance. Our live ESG dashboard can be used to scenario plan, map out ESG strategy and tell the ESG story to stakeholders.
AI helps with the process of ingesting, analysing and distributing data as well as offering predictive abilities and assessing trends in the ESG space. Leading Point is helping our AI startup partnerships adapt their technology to pursue this new opportunity, implementing these solutions into investment firms and supporting them with the use of the technology and data management.
We offer a specialised and personalised service based on firms’ ESG priorities. We harness the power of technology and AI to bridge the ESG data gap, avoiding ‘greenwashing’ data trends and providing a complete solution for organisations.
Leading Point's AI-implemented solutions decrease the time and effort needed to monitor current/past scandals of potential investments. Clients can see the benefits of increased output, improved KPIs and production of enhanced data outputs.
Implementing ESG regulations and providing operational support to improve ESG metrics for banks and other financial institutions. Ensuring compliance by benchmarking and disclosing ESG information, in-depth data collection to satisfy corporate reporting requirements, conducting appropriate investment and risk management decisions, and to make disclosures to clients and fund investors.
Regulatory Risk: Getting away from Whack-a-Mole
Senior Management is under more pressure than ever to demonstrate compliance and risk-sensitive decision making - but the process by which they do it is straining under the sheer number and weight of obligations to manage.
36% of fines handed out by the FCA over the last 3 years - over a third - have been for failings related to management and control (PRIN 3)*. With an average penalty of £24 million firms cannot afford to be lax in this. Transparency of their firm’s systems and controls continues to be vital for leaders at Board level and within Senior Management Functions to ensure that their business is compliant and within risk tolerances.
Increasingly, during the ongoing pandemic, regulators expect comprehensive, responsible, and tangible governance and control to be operated by regulated firms. Creating transparency of firms’ regulatory activity across the business paramount. Not just for leaders at Board and Senior Management Functions levels (SMFs) but also in the supporting infrastructure within Compliance, Operations, Technology, Finance, Legal, and HR.
In their recent Joint Statement for Firms, the UK regulators outlined that firms must:
“Develop and implement mitigating actions and processes to ensure that they continue to operate an effective control environment: in particular, addressing any key reporting and other controls on which they have placed reliance historically, but which may not prove effective in the current environment. .. Consider how they will secure reliable and relevant information, on a continuing basis, in order to manage their future operations.”**
Joint statement by the Financial Conduct Authority (FCA), Financial Reporting Council (FRC) and Prudential Regulation Authority (PRA), 26th March 2020
‘Securing reliable and relevant information’ is harder than it sounds. The information required for this is frequently cobbled together in PowerPoint, Excel or other tools from a wide variety of disparate sources. This is inefficient and time intensive, and is subject to inconsistencies. Information may be out of date by the time it is produced, and often does not meet the level of detail required by the various audiences.
More than that, Senior Managers lack a consolidated view of their regulatory risk across their business. This is difficult to achieve given the number of areas they need to monitor, ongoing regulatory change, and the pace of digital transformation. Managers are often spending more time piecing together a picture of their overall regulatory ‘health’ and fighting fires than they are developing the business.
Compliance issues become like Whack-A-Mole, as soon as one gets whacked, another one pops up, and then another. Senior Management are effectively blindfolded holding the ‘mole hammer’ and have to ask a business analyst or a compliance officer “are there any moles today?” and “what do I hit?”.
These regulatory moles are not common or garden business problem moles. There may be hundreds of moles to whack at any given time. As a result, managers need the ability to triage the reports of mole sightings to decide which is most pressing. Which is most likely to ruin his or her lawn? Is it the Sanctions Breach mole, the Data Protection mole or Transaction Reporting mole?
Not only are there many of them - you need to keep records of which ones you’ve whacked and why. At some point you’ll need to evidence why you didn’t whack the Sanctions Breach mole immediately and provide the context for that decision. If you fail to whack enough of them, or the right ones, your business could be fined, or worse, you personally could end up in court.
This is a much more pressing issue due to the level of personal accountability, and broadened personal liability, introduced by the Senior Managers and Certification Regime (SM&CR). The SM&CR, which came into force on 9th December 2019, overhauled the Approved Persons Regime for individuals working in UK financial services firms. Placing more stringent requirements on senior managers to take responsibility for their firms’ activities through a ‘Duty of Responsibility’ to take ‘reasonable steps’ to prevent or stop regulatory breaches.
As the FCA Handbook states in their “Specific guidance on individual conduct rules” (COCON 4.2) addressed to Senior Managers: “SC2: You must take reasonable steps to ensure that the business of the firm for which you are responsible complies with the relevant requirements and standards of the regulatory system.”***
We believe that one of these ‘Reasonable Steps’ is having appropriate reporting to achieve a clear view of the ‘Regulatory Health’ of their business and their risk points. Firms and Senior Managers need the ability to:
- Capture key regulatory risk metrics
- Link them to the appropriate compliance monitoring data
- Put those risk metrics into context across the business
- Generate a consolidated view of the business’ regulatory health and risk points
- Make it accessible & easily understandable to the relevant managers
- Make it ‘persistent’ over time to and allow ‘point in time’ views of risk levels
A solution that could a) take existing and live compliance data b) isolate the risk metrics that really ‘matter’, and c) present them in context across regulations and business areas is really needed for Senior Managers to have a picture of their overall risk.
Senior Management should know where the regulatory moles are - without having to ask. Rather than having to review reams of documentation, it could allow managers a more holistic and focused view of regulatory risk across their business, as well as save time and resource spent creating, managing, and reviewing PowerPoints. Knowing what to look for is half the battle after all.
Don’t let the moles ruin your lawn.
References
1. Leading Point analysis of FCA fines related to PRIN 3 Management and control: A firm must take reasonable care to organise and control its affairs responsibly and effectively, with adequate risk management systems.” FCA Principles for Business https://www.handbook.fca.org.uk/handbook/PRIN/2/?view=chapter
3. “Specific guidance on individual conduct rules” (COCON 4.2) addressed to Senior Managers: https://www.handbook.fca.org.uk/handbook/COCON/4/2.html
On July 14th, experts from banks, hedge funds and market infrastructure providers will discuss how financial institutions can create transparency and insights from their regulatory risk data, and Leading Point will introduce their new industry-leading regulatory risk data system SMART_Dash.
Panellists will discuss:
- The challenges of internal regulatory oversight that all financial services firms are facing
- How businesses can create a consolidated view of their regulatory risk
- The ways that regulatory monitoring data can be more accessible
- An introduction to SMART_Dash; a revolutionary tool providing regulatory risk reassurance
*Regulatory Risk, not moles
Join our webinar to learn more about how to create transparency and insights from regulatory risk data
Senior Management are effectively blindfolded holding the ‘mole hammer’ and have to ask a business analyst or a compliance officer “are there any moles today?” and “what do I hit?”.
36% of fines handed out by the FCA over the last 3 years - over a third - have been for failings related to management and control (PRIN 3).
"[Firms must] Consider how they will secure reliable and relevant information, on a continuing basis, in order to manage their future operations."
"firms need to ensure that their cloud-based operating models are not only safe and secure, but address the capabilities required for operational resilience testing. Investment in frameworks and data analytics that can support these capabilities are essential"
Thushan Kumaraswamy
Head of Solutions
Architecture lead with over 20 years’ experience helping the world’s biggest financial services providers in capital markets, banking and buy-side to deliver practical business transformations in client data, treasury, sales, operations, finance and risk functions, and major firm-wide efficiency initiatives. Mastery in business and technical architecture, with significant experience in end-to-end design, development and maintenance of mission critical systems in his early career. Specialities – business and technical architecture leadership, data warehousing, capital markets, wealth management, private banking.
Rajen Madan
Founder & CEO
Change leader with over 20 years’ experience in helping financial markets with their toughest business challenges in data, operating model transformation in sales, CRM, Ops, Data, Finance & MI functions, and delivery of complex compliance, front-to-back technology implementations. Significant line experience. Former partner in management consulting leading client solution development, delivery and P&L incl. Accenture. Specialities – Operating Models, Data Assets, Compliance, Technology Partnerships & Solutions in Capital Markets, Market Infrastructure, Buy-Side, Banking & Insurance.
What if business operations could be more like Lego?
Financial services (FS) professionals from 30+ organisations tuned in to our inaugural webinar last week “What if business operations could be more like Lego?” to hear the challenges that COO and Heads of Change face in changing their business operating models and how we might break through the barriers. A summary of key takeaways from the discussion are presented below. See the webinar recording here
The importance of ‘Know Your Operating Model’
FS firms are under renewed pressure to rethink their operating models; competitive pressure, raised consumer expectations, and continuous regulatory requirements mean constant operating model re-think and change. Yet most firms are stuck with theoretical target operating models that lack a plan, a way to measure performance and progress, or a business case. As a result, only 25% of investors are confident strategic digital transformation will be effective.**
Innovation is hindered as firms struggle to overcome significant technical debt to implement new technology (e.g. automation, AI, cloud etc.) while effectively using budget tied up in high operating costs. Indeed, 80% of technology spend in organisations is focused on legacy systems and processes, while only 20% of analytics insights deliver business outcomes and 80% of AI projects “remain alchemy, run by wizards”***
Insufficient business understanding means lost opportunities, wasteful spends & risk – if you don’t understand your business well enough, you will be exposing yourself to risks and lost opportunities.
The barriers to business understanding
Firms current approaches to business operations and change are not fit for purpose.
Insight Gap in the Boardroom: Experts with specialist toolkits are needed to structure and interpret most business information. Management’s understanding of the business is often directly related to the ability of their analytical teams to explain it to them. Most firms are still stuck with an overload of information without insights, without the right questions being asked.
Cultural Challenge: Many execs still think in terms of headcount and empire building rather than outcomes, capabilities, and clients.
Misaligned metrics: Metrics are too focused on P&L, costs and bonuses! Less on holistic organisation metrics, proof points and stories.
Complexity makes it difficult to act… Most enterprises suffer from excessively complicated operating models where the complexity of systems, policies, processes, controls, data and their accompanying activities make it difficult to act.
…and difficult to explain: Substantiating decisions to stakeholders, regulators or investors is an ongoing struggle, for both new and historic decisions.
If you can't measure it, you can't manage it: Inconsistent change initiatives without performance metrics compound errors of the past and mean opportunities for efficiency gains go unseen.
How can we break through these barriers?
Business insight comes from context, data and measurement: How the building blocks of the business fit together and interact is essential to the ‘what’ and ‘how’ of change, and measurement is key to drive transparency and improved behaviours.
Operating model dashboards are essential: Effective executives either have extremely strong dashboards underpinning their decisions or have long standing experience at the firm across multiple functions and get to “know” their operating mode innately. This is a key gap in most firms. 50% of attendees chose improved metrics & accessibility of operating model perspectives as priority areas to invest in.
Less is more: Senior managers should not be looking at more than 200 data points to run and change their business. Focusing on the core and essential metrics is necessary to cut through the noise.
The operating model data exists, it should now be harvested: The data you need probably already exists in PowerPoint presentations, Excel spreadsheets and workflow tools. Firms have struggled to harvest this data historically and automate the gathering process. We demonstrated how operating model data can be collected and used to create insights for improved decision-making using the modellr platform.
Culture change is central: Culture was voted by attendees as the #1 area to invest in, in order to improve business decision-making. Organisational culture is a key barrier to operating model change. A culture that incentivises crossing business silos and transparency will create benefits across the enterprise.
Client-driven: Clients are driving firms to more real-time processing along with the capability to understand much more information. Approaches that combine human intelligence with machine intelligence are already feasible and moving into the mainstream.
Get comfortable with making decisions with near perfect information: Increasingly executives and firms need to get comfortable with “near perfect” information to make decisions, act and deliver rapid business benefits.
Future Topics of Interest
Regulatory Reassurance: Regulators continue to expect comprehensive, responsible and tangible governance and control from Senior Managers. How can firms keep up with their regulatory obligations in a clear and simple way?
Environmental, Social & Governance (ESG): An increasingly-popular subject, ESG considers the impact of businesses on the environment and society. ESG metrics are becoming more important for investors & regulators and firms are looking for consistent ways to measure performance and progress in ESG metrics.
Operating Model-as-a-Service: As well as managing business operations themselves, firms need to monitor the models that describe those operations; their current state, their target state and the roadmap between the two. Currently, this is often done with expensive PowerPoint presentations that are usually left in cupboards and ignored because they are not “live” documents. Metrics around the operating model can be captured and tracked in a dashboard.
Anti-Financial Crime (AFC): Money laundering, terrorist financing, fraud, sanctions, bribery & corruption; the list of ways to commit financial crime through FS firms grows by the day. How can firms track their AFC risk levels and control effectiveness to see where they need to strengthen?
Information Security: With the huge volume of data that firms now collect, process & store, there are more and more risks to keep that data secure and private. Regulations like GDPR can impose very large fines on firms that break those regulations. Industry standards, such ISO 27001, help improve standards around information security.
*,** Oliver Wyman, 2020, The State Of The Financial Services Industry
*** Gartner, 2019, Our Top Data and Analytics Predicts for 2019
Reimagining trading platform support: Who's supporting you through turbulent times?
Trading platform support is, and has been, going through some heavy changes. It’s a changing world we live in and even putting the current situation to one side (we know it’s difficult but let’s try) it’s worth noting how cost reduction, market consolidations, and changes in approach, etc. have changed the landscape for how trading platforms are supported.
Good front line support for trading platform functionality is now more difficult to access and slower to respond resulting in fewer issues actually being resolved.
Changes in focus from vendors has meant the trading industry has had to come up with, let’s face it, a compromise, to ensure their businesses can continue to operate ‘as normal’. There are many new normals across all industries and sectors at present, but the trading world is highly arcane in nature and therefore any change is difficult for traders and salespeople alike. This has translated into moves towards other models like ‘Live Chat’ style support, which some find impersonal, with fewer experienced people showing up regularly at client sites.
At the sharp end this can mean less voice support and a reduction in face to face support resulting in declining reassurance for users from regular contact with the ‘floorwalkers’. Some trading platform users have found that trading support has been neglected and their experience has suffered as a consequence.
For instance, a Waters Technology article, published last year, reported one Fidessa user citing difficulties with issue resolution:
“It seems like they’ve lost the ability to distinguish between a general issue and an urgent issue that needs to be resolved because it’s putting our clients at risk. We’ve had some issues that have been sitting with them for months.”
Obviously this is a sub-optimal ongoing predicament to be in. Whether due to cost savings, staff attrition rates or other reasons – the provision of first line support has deteriorated.
Even so, the cost of support to a trading firm remains constant in real terms. But in terms of what they get in return, it effectively becomes an added overhead translating to something with a diminishing return.
Added to these ongoing, and somewhat reluctantly accepted concerns, new uncertainties are pushing themselves to the forefront of users minds. The big one currently of course are the changes companies and staff are having to make now to their working arrangements in relation to the current climate and the need to maintain a distributed workforce.
Uncertainties around this mean that some in this space now acknowledge a real need for flexibility and better business continuity planning and scalability options (there have been significant spikes in volumes and volatility) in the approach to providing support for users. One just needs to look at the increasing number of LinkedIn or Facebook posts of people attempting to replicate their office desk at home to see the level of impact.
All of the above factors appear to be leading to a dawning realisation for many trading platform users for two necessary changes:
- A higher degree of self-sufficiency for navigating a platform and making full use of its features.
- Fast and reliable turnaround for resolving complex issues and being trained in new functionality without the necessity to call upon a fixed cost resource pool.
So what is the obstacle here?
Think about applications like Word or Excel. How many people who regularly use applications such as these are proficient in just enough to enable them to carry out their daily job? Many of these people are probably utilising less than ten percent of what the application offers and therefore unable to identify avoidable bottlenecks and efficiency gains no matter how simple to implement – 90% of the potential benefits remain unused, an ‘unknown unknown’.
With such a wealth of functionality offered, knowing what *really* matters requires an understanding of both the application and your specific needs.
The same can be said for trading functionality; untapped opportunities for improved workflows are lying undiscovered and unutilised before users’ eyes. Comprehensive support and training in existing and new functionality can pave the way for users to discover that potential including, dare we say, the opportunity of alpha generation due to the possibility of speed of use through innate familiarity.
Communication and tailored collaboration with knowledgeable and experienced support teams is essential. Targeted, independent and focused front line support available from experienced outsourced providers presents a viable support proposition for platform users, wherever you sit in the organisation.
At Leading Point we are not only able to react to issues quickly but also know the information you are looking for (often before you need it) that will make a real difference to your daily trading platform experiences. With an innate ability to speak your ‘language’ we can provide seamless communication. All of this underpinned by an always available service when you and your users need it most.
- Imagine an innovative trading support experience comprising an equally innovative commercial model enhancing an entire trading platform experience.
- Imagine the knowledge your users can benefit from through such a collaboration and the degree to which that benefit is passed on to clients
- Imagine, through the unlocking of that untapped potential, your regular users becoming super users
The time for change is NOW. If you’d like to get in touch, we would be delighted to tell you more about the potential benefits to you and your firm.
Untapped opportunities for improved workflows are lying undiscovered and unutilised before users’ eyes.
Good front line support for trading platform functionality is now more difficult to access and slower to respond resulting in fewer issues actually being resolved.
“It seems like they’ve lost the ability to distinguish between a general issue and an urgent issue that needs to be resolved because it’s putting our clients at risk.”
Excel Ninjas & Digital Alchemists – Delivering success in Data Science in FS
In February 150+ data practitioners from financial institutions, FinTech, academia, and professional services joined the Leading Point Data Kitchen community and were keen to discuss the meaning and evolving role of Data Science within Financial Services. Many braved the cold wet weather and made it across for a highly productive session interspersed with good pizza and drinks.
Our expert panellists discussed the “wild” data environment in Financial Services inhabited by “Excel Ninjas”, “Data Wranglers” and “Digital Alchemists”. But agreed that despite the current state of the art being hindered by legacy infrastructure and data siloes there are a number of ways to find success.
Here is the Data Kitchen’s ‘Recipe’ for delivering success in Data Science in Financial Services:
1. Delivery is key – There is a balance to strike between experimentation and delivery. In commercial environments, especially within financial services there is a cost of failure. ROI will always be in the minds of senior management, and practitioners need to understand that is the case. This means that data science initiatives will always be under pressure to perform, and there will be limits on the freedom to just experiment with the data.
2. Understand how to integrate with the business – Understanding what ‘good’ delivery looks like for data science initiatives requires an appreciation of how the business operates and what business problem needs to be solved. Alongside elements of business analysis, a core skill for practitioners is knowing how to ‘blend in’ with the rest the business – this is essential to communicate how they can help the business and set expectations. “Data translators” are emerging in businesses in response.
3. Soft skills are important – Without clear articulation of strategy and approach, in language they can understand, executives will often either expect ‘magic’ or will be too nervous to fully invest. Without a conduit between management and practitioners many initiatives will be under-resourced or, possibly worse, significantly over-resourced. Core competencies around stakeholder and expectation management, and project management is needed from data practitioners and to be made available to them.
4. Take a product mindset – Successful data science projects should be treated in a similar way to developing an App. Creating it and putting it on the ‘shelf’ is only the beginning of the journey. Next comes marketing, promotion, maintenance, and updates. Many firms will have rigorous approaches to applying data quality, governance etc. on client products, but won’t apply them internally. Many of the same metrics used for external products are also applicable internally e.g. # active users, adoption rates etc. Data science projects are only truly successful when everyone is using it the way it was intended.
5. Start small and with the end in mind – Some practitioners find success with ‘mini-contracts’ with the business to define scope and, later, prove that value was delivered on a project. This builds a delivery mindset and creates value exchange.
6. Conduct feasibility assessments (and learn from them) – Feasibility criteria need to be defined that take into account the realities of the business environment, such as:
- Does the data needed exist?
- Is the data available and accessible?
- Is management actively engaged?
- Are the technology teams available in the correct time windows?
If you run through these steps, even if you don’t follow through with a project, you have learned something – that learning needs to be recorded and communicated for future usage. Lessons from nearly 100+ use cases of data science in financial services and enterprises, suggest that implementing toll-gates for entry and exit criteria is becoming a more mature practice in organisations.
7. Avoid perfection - Sometimes ‘good’ is ‘good enough’. You can ‘haircut’ a lot of data and still achieve good outcomes. A lot of business data, while definitely not perfect, is being actively used by the business – glaring errors will have been fixed already or been through 2-3 existing filters. You don’t always need to recheck the data.
8. Doesn’t always need to be ‘wrangled’ – Data Scientists spend up to 80% of time on "data cleaning" in preparation for data analysis but there are many data cleansing tools now in the market that really work and can save a lot of time (e.g. Trifacta). Enterprises will often have legacy environments and be challenged to connect the dots. They need to look at the data basics – an end to end data management process, the right tools for ingestion, normalisation, analysis, distribution and embedding outputs as part of improving a business process or delivering insights.
Our chefs believed Data Science will evolve positively as a discipline in the next three years with more clarity on data roles, a better qualification process for data science projects, application of knowledge graphs, better education and cross pollination of business and data science practitioners and the need for more measurable outcomes. The lessons from failures are key to make the leap to data-savvy businesses.
Just a quick note to say thank you for your interest in The Data Kitchen!
We had an excellent turn out of practitioners from organisations including: Deutsche Bank, JPMorgan, HSBC, Schroders, Allianz Global Investors, American Express, Capgemini, University of East London, Inmarsat, One corp, Transbank, BMO, IHS Markit, GFT, Octopus Investments, Queen Mary University, and more.
And another Thank You to our wonderful panellists!
- Peter Krishnan, JP Morgan
- Ben Ludford, Efficio
- Louise Maynard-Atem, Experian
- Jacobus Geluk, Agnos.ai
…And Maître De – Rajen Madan, Leading Point FM
We would like to thank our chef’s again and to all participants for sharing plenty of ideas on future topics, games and live solutions.
LIBOR Signals Need for New Approaches to Legal Data
The Scope of LIBOR Remediation is the Problem
Time is now of essence – with the end of 2021 deadline looming, financial institutions need to reduce their ‘stock’ of legacy LIBOR contracts to a minimum as a matter of priority, writes Rajen Madan, CEO, Leading Point.
The challenge is of course colossal. Firms need to find every reference to IBORs embedded in every contract they hold; update each contract with fallback provisions / reflect the terms of the alternative reference rate they are migrating to; and communicate the results with clients.
LIBOR’s retirement potentially impacts over $350 trillion of contracts and requires all LIBOR transactions (estimated at over 100 million documents) to be examined and most likely repapered. LIBOR is embedded in every asset class – mortgages and retail loans, to commodities, derivatives, bonds and securities.
It’s estimated that large banks may be exposed to more than 250,000 contracts directly referencing LIBOR maturing after 2021, and indirectly exposed to many thousands more embedded in servicing activities, supplier agreements and such.
Only 15 percent of Financial Institutions are ready to deal with this volume of contract remediation, deal restructuring, and repapering activities required for the scale of their legacy contract back-book; 14 of the world’s top banks expect to spend more than $1.2 billion on the LIBOR transition.
Firms that have comprehensive visibility of their legal contract information via retained structured data, can avoid 80 percent of the typical repapering process, and focus their efforts on the remaining, critical, 20 percent.
LIBOR Repapering Not a ‘Find and Replace’
The repapering of contracts isn’t as straightforward as a ‘Find and Replace’ on legal terminology referencing LIBOR.
Risks are many including conduct, legal, prudential and regulatory. Consider ‘conduct’ risk. In the UK, the Treating Customers Fairly (TCF) regime is particularly concerned with how customers are affected by firms’ LIBOR transition plans. Before contracts can be updated, firms will need to ensure that LIBOR linked products and services have ‘fair’ replacement rates that operate effectively.
Similarly, there’s prudential risk. When the underlying contracts change, firms may find that the instruments they rely on for capital adequacy purposes may no longer be eligible, potentially even resulting in a sudden drop in a bank’s capital position. Similarly, there are several Counterparty Credit, Market, Liquidity, and Interest Rate Risks that will need to be reflected in firms’ approaches.
LIBOR is proving to be a real impetus for financial institutions to use technology that, to be honest, has been available in the marketplace for a long time now.
Mindset Change is Needed to Manage Legal Data
Most historic repapering exercises have involved hastily identifying the documents impacted, outsourcing the difficult issues to law firms (at huge cost) and throwing manpower (again at substantial cost) at the problem to handle the contract updates and communications with counterparties. The exact same process has been repeated for every repapering project. Despite the substantial costs, many financial institutions still don’t meet the deadline. MiFID II is an example.
With ample evidence of regulators continually tightening their grip on financial institutions through reform – alongside an increasingly dynamic global business environment (e.g. LIBOR, Brexit) – it is time organisations acknowledged and accepted repapering as a ‘business as usual’ activity.
A change in mindset and a smarter approach is needed to manage legal data. Financial institutions need to ensure that LIBOR or indeed any future business repapering exercise does not compromise client well-being or negatively impact client experience. For instance, to accurately model the financial risk firms’ portfolios are exposed to via LIBOR when transitioning to a new rate, they need a way to directly link, say, multiple cash and derivative contracts to a single client. Furthermore, in an environment where most firms are product driven, the scenario of multiple repetitive communications, requests for information and re-papering contract terms looms on the horizon for firms’ customers.
It is heartening to see that LIBOR is beginning to pique the interest of financial institutions to develop a long-term vision to create smarter capabilities that will deliver business advantages in the future.
Stephanie Vaughan, Global Legal AI Practice Director at iManage RAVN and ex-Allen & Overy, recently observed, “LIBOR is proving to be a real impetus for financial institutions to use technology that, to be honest, has been available in the marketplace for a long time now. While they may have dabbled with it in the past, due to the scale of the LIBOR remediation and the constantly changing regulatory challenges, it has finally hit home that such projects are a drain on resources and are delivering no business value.”
Financial institutions have started every repapering project (e.g. MiFID II, Dodd Frank, Margin Rules) from scratch including going through the entire process of determining the clients, what the terms of engagement are, when the contracts expire and so on.
Technology Can Make Repapering ‘Business as Usual’
A strategic approach to managing legal data requires all stakeholders in a financial institution to come on board – from business units and the compliance department through to legal operations and the General Counsel. This is instrumental to ensuring genuine cross-functional recognition and support for strategic directional change.
Financial institutions need to build a strong, technology-supported foundation for remediation projects. Thus far, financial institutions have started every repapering project (e.g. MiFID II, Dodd Frank, Margin Rules) from scratch including going through the entire process of determining the clients, what the terms of engagement are, when the contracts expire and so on.
Hereafter, with LIBOR and Brexit, extracting, classifying, storing and maintaining all these data points as structured, base level information on customers on a single technology platform, will provide institutions with capabilities to quickly understand their exposure, assign priorities and flexibly make contractual changes in tune with evolving requirements.
This approach is proven. Firms that have comprehensive visibility of their legal contract information via retained structured data, can avoid 80 percent of the typical repapering process, and focus their efforts on the remaining, critical, 20 percent. Financial institutions will then also be well poised to take advantage of new bolt on capabilities that leverage artificial intelligence for application to specific use-cases – which in turn can deliver business value from contract search, contract classification, clause management, to real time analytics, contract generation and integration with operational, risk and compliance systems.
The opportunity with more effective legal data management is huge and realisable. Building and incrementally strengthening capability through the strategic and proactive use of technology is potentially the only way for financial institutions to adapt to their new regulatory and business environment.
The repapering of contracts isn’t as straightforward as a ‘Find and Replace’ on legal terminology referencing LIBOR.
LIBOR: Manual Approaches are no Longer Enough to Manage FS Legal Data
The transition away from LIBOR is the biggest contract remediation exercise in Financial Services history – and firms are under prepared.
As the Bank of England and FCA lays out in bold font, in their January 2020 letter to CEOs, “LIBOR will cease to exist after the end of 2021. No firm should plan otherwise.”[1] As a result, Financial Institutions have very little time to reduce their “stock of legacy LIBOR contracts to an absolute minimum before end-2021”.
The challenge is this:
1. Firms have to find every reference to IBORs embedded in every contract they hold.
2. Update each contract with fallback provisions or to reflect the terms of the alternative reference rate they are migrating to.
3. Communicate the results with clients
This is much easier said than done due to the sheer scale of the task.
LIBOR’s retirement has the potential to impact over US$ 350 trillion of contracts and will require all LIBOR transactions (estimated at over 100 million documents) to be examined and most likely repapered. LIBOR is embedded in far more than just derivative contracts. Every asset class is affected; from mortgages and retail loans, to commodities, bonds or securities. The resolution of Lehman Brothers after 2008 gives some idea of the scale of the repapering effort for each firm – Lehman was party to more than 900,000 derivatives contracts alone.
The scope of the problem is part of the problem. Hard numbers are difficult to come by as no-one really knows exactly what their exposure is, or how many contracts they need to change.
Current estimates say large banks’ may be exposed to more than 250,000 contracts directly referencing LIBOR maturing after 2021, and indirectly exposed to many thousands more embedded in servicing activities, supplier agreements or more.
Only 15% of Financial Institutions are ready to deal with this volume of contract remediation, deal restructuring, and repapering activities required for the scale of their legacy contract back-book.[2] Fourteen of the world’s top banks expect to spend more than $1.2 billion on the LIBOR transition[3].
To approach the LIBOR transition manually will likely require years of man-hours and cost millions of dollars, with significant potential for human error
There are a wide variety of risks to consider.
But it’s not as straightforward as a ‘Find and Replace’ on legal terminology referencing LIBOR. Firms face huge operational, conduct, legal and regulatory risk arising from both the difficulties in managing the vast volumes of complex client contractual documentation but also the downstream impacts of that documentation having been changed.
Conduct Risk: In the UK, the Treating Customers Fairly (TCF) regime is particularly concerned with how customers are affected by firms’ LIBOR transition plans. Before contracts can be updated, firms will need to ensure that LIBOR linked products and services have ‘fair’ replacement rates that operate effectively.[1] Firms will also need to ensure that any changes made are applied across the entire customer ‘class’ to comply with TCF rules and avoid preferential treatment issues.
Legal Risk: There is a huge amount of legal risk arising from disputes in what interest rates should be paid out in amended agreements referencing alternative reference rates.[2] The ISDA protocol expected to be published in Q2 2020 should help with, but not solve, these problems.[3]
This is not to mention the legacy contracts that cannot legally be converted or amended with fallbacks – named by Andrew Bailey at the FCA as the ‘tough legacy’.[4] The UK Working Group on Sterling Risk Free Reference Rates (RFRWG) is due to publish a paper on ‘tough’ legacy contracts in the second half of Q1 2020.[5]
The realism of firms’ assessments of the number of contracts requiring renegotiation should be considered a legal risk in itself – a realised 10% increase in this number would likely incur serious, additional legal fees.
Prudential Risk: When the underlying contracts change, firms may find themselves in a position where suddenly the instruments they rely on for capital adequacy purposes may no longer be eligible - “This could result in a sudden drop in a bank’s capital position.” [6] For similar reasons, there are a number of Counterparty Credit, Market, Liquidity, and Interest Rate Risks that will need to be reflected in firms’ approaches.
Regulatory Risk: Regulators are closely monitoring firms’ transition progress – and they are not happy with what they are seeing. Financial Policy Committee (FPC) stated in January, 2020, has made clear that they are ‘considering’ the supervisory tools that authorities could use to “encourage the reduction in the stock of legacy LIBOR contracts to an absolute minimum before end-2021.”[7] This is regulatory code for ‘we will either fine or increase the capital requirements for firms we judge to be dropping the ball’. The PRA and FCA laid out their expectations for the transition in June 2019 – this is required reading for any LIBOR transition project manager.[8]
It’s not as straightforward as a ‘Find and Replace’ on legal terminology referencing LIBOR
What this means for firms is that they need:
1. The capability to quantify their LIBOR exposure – Firms need a good understanding of their LIBOR contractual exposure that can quantify a) firms’ contractual population (i.e. which documents are affected) b) the legal, conduct and financial risk posed by the amendment of those documents
2. The ability to dynamically manage and track this exposure over time – As strategies evolve, the regulatory environment changes, and new scenarios develop, so will firms’ exposure to LIBOR change. Without good quality analytics that can track this effectively, in the context of this massive change project, firms will be strategically and tactically ‘flying blind’ in the face of the massive market shifts LIBOR will bring about.
3. The capability to manage documentation - Jurisdictional, product, or institutional differences will necessitate large client outreach efforts to renegotiate large populations of contracts, manage approvals & conflict resolution, while tracking interim fall-back provisions and front office novation of new products to new benchmarks.
Accomplishing the above will require enterprise-wide contract discovery, digitisation, term extraction, repapering, client outreach and communication capabilities – and the ability to tie them all together in a joined-up way.
To approach the LIBOR transition manually will likely require years of person-hours and cost millions of dollars, with significant potential for human error.
Accomplishing the above will require enterprise-wide contract discovery, digitisation, term extraction, repapering, client outreach and communication capabilities – and the ability to tie them all together in a joined-up way
LIBOR cannot be treated as ‘just one more’ repapering exercise.
Firms are continually hit with new requirements which require the update, negotiation and amendment of client contracts.
The reaction is always the same: Scramble to identify the documents impacted, outsource the thornier problems to external legal, and hire huge teams of consultants, remediation armies and legal operations to handle the contract updates and communications with counterparties.
Once complete - often months past the deadline - everyone stands down and goes home. Only to do the same thing again next year in response to the next crisis. While this gets the job done, there are number of problems with this project by project approach:
1. It’s inefficient: Vast amounts of time (and money) is spent just finding the documents distributed around the business, often in hard copy, or locked away in filing cabinets.
2. It’s expensive: External legal, consultants and remediation shops don’t come cheap – especially when the scope of the project inevitably expands past the initial parameters.
3. It’s ineffective: Little to no institutional knowledge is retained of the project, no new processes are put in place, and documents continue to get locked away in filing cabinets - meaning when the time comes to do it again firms have to start from scratch.
When you look at the number of major repapering initiatives over the past 10 years the amount of money spent on repapering projects is monumental. In the EU alone, regulations such as MiFID II, EMIR, GDPR, PPI, FATCA, Brexit and AIFMD have each required a huge repapering project. In 2020, LIBOR, Initial Margin Rules and SFTR will each require contract remediation programmes.
Doing ‘just another’ repapering exercise for LIBOR is a risky mistake. There is a better way.
Smarter data management and enabling tech solutions can help identify, classify and extract metadata from the huge volumes of LIBOR impacted documents at speed. The ability to extract and store contractual information as structured information at this scale allows firms’ the essential capabilities to understand and track their LIBOR exposure, assign priorities and maintain flexibility in a changing situation.
Firms that have fuller visibility of their legal contract information, retained as structured data, can avoid 80% of the typical repapering process, and focus their efforts on the remaining, critical, 20%.[1] The time spent manually identifying contractual needs, can be reallocated to the areas that matter, freeing up legal resource, budget, and project timelines – while simultaneously improving client relationships.
This should not be seen just as a repapering enabler, but a strategic capability. The opportunities afforded through data mining firms’ contractual estate for analytics are vast.
Doing ‘just another’ repapering exercise for LIBOR is a risky mistake. There is a better way
One possibility is the ability to connect contracts directly to trades. To accurately model the financial risk firms’ portfolios are exposed to via LIBOR when transitioning to a new rate, they will need a way to directly link, for example, multiple cash and derivative contracts to a single client. Firms are still a long way from this capability – but there are a growing number of sophisticated artificial intelligence solutions that can begin to address these types of use-cases.
Firms that build these capabilities now will materially reduce their risk exposures, improve liquidity and funding, build trust with their clients and be much better equipped to meet other pressing regulatory requirements such as Brexit, SFTR, CRD 5/6, Initial Margin (IM) rules, QFC and more.
[1] ‘Next steps on LIBOR transition’, January 2020, FCA & PRA https://www.fca.org.uk/publication/correspondence/dear-smf-letter-next-steps-libor-transition.pdf
[2] 2019 LIBOR Survey: Are you ready to transition?, September 2019, Accenture. https://www.accenture.com/_acnmedia/109/Accenture-2019-LIBOR-Survey-fixed.pdf#zoom=50
[3] ‘The end of Libor: the biggest banking challenge you've never heard of’, October 2019, Reuters.
[4] Firms will also need to consider whether any contract term they may rely on to amend a LIBOR-related product is fair under the Consumer Rights Act 2015 (the CRA) in respect of consumer contracts. FG18/7: Fairness of variation terms in financial services consumer contracts under the Consumer Rights Act 2015 contains factors that firms should consider when thinking about fairness issues under the CRA when they draft and review unilateral variation terms in their consumer contracts. https://www.fca.org.uk/markets/libor/conduct-risk-during-libor-transition
[5] Litigation risks associated with Libor transition: https://collyerbristow.com/longer-reads/litigation-risks-associated-with-libor-transition/
[6] UK Working Group on Sterling Risk-Free Reference Rates (RFR WG) 2020 Top Level Priorities. https://www.bankofengland.co.uk/-/media/boe/files/markets/benchmarks/rfr/rfrwgs-2020-priorities-and-milestones.pdf?la=en&hash=653C6892CC68DAC968228AC677114FC37B7535EE
[7] LIBOR: preparing for the end, https://www.fca.org.uk/news/speeches/libor-preparing-end
[8] UK Working Group on Sterling Risk-Free Reference Rates (RFR WG) 2020 Top Level Priorities. https://www.bankofengland.co.uk/-/media/boe/files/markets/benchmarks/rfr/rfrwgs-2020-priorities-and-milestones.pdf?la=en&hash=653C6892CC68DAC968228AC677114FC37B7535EE
[9] Letter from Sam Woods: The prudential regulatory framework and Libor transition, Bank of England, https://www.bankofengland.co.uk/-/media/boe/files/prudential-regulation/letter/2019/prudential-regulatory-framework-and-libor-transition.pdf?la=en&hash=55018BE92759217608D587E3C56C0E205A2D3AF4
[10] ‘Next steps on LIBOR transition’, January 2020, FCA & PRA https://www.fca.org.uk/publication/correspondence/dear-smf-letter-next-steps-libor-transition.pdf
[11] ‘Firms’ preparations for transition from London InterBank Offered Rate (LIBOR) to risk-free rates (RFRs): Key themes, good practice, and next steps.’, June 2019, FCA & PRA https://www.bankofengland.co.uk/-/media/boe/files/prudential-regulation/publication/2019/firms-preparations-for-transition-from-libor-to-risk-free-rates.pdf?la=en&hash=EA87BD3B8435B7EDF25A56C932C362C65D516577
[12] MiFID II – the long tail of legal documentation repapering, https://www.fintechfutures.com/2018/04/mifid-ii-the-long-tail-of-legal-documentation-repapering/
Artificial Intelligence & Anti-Financial Crime
Leading Point Financial Markets recently hosted a roundtable event to discuss the feasibility of adopting Artificial Intelligence (AI) for Anti-Financial Crime (AFC) and Customer Lifecycle Management (CLM).
A panel of SMEs and an audience of senior execs and practitioners from 20+ Financial Institutions and FinTechs discussed the opportunities and practicalities of adopting data-driven AI approaches to improve AFC processes including KYC, AML, Payment Screening, Transaction Monitoring, Fraud & Client Risk Management.
“There is no question that AI shows great promise in the long term – it could transform our industry…” Rob Gruppetta, Head of the Financial Crime Department, FCA, Nov 2018
EXECUTIVE SUMMARY
AFC involves processing and analysing vast volume and variety of data; it’s a challenge to make accurate & timely decisions from it.
Industry fines, increasing regulatory requirements, a steep rise in criminal activities, cost pressures and legacy infrastructures is putting firms under intense pressure to up their game in AFC.
90% expressed the volume and quality of data as a top AFC/CLM challenge for 2019.
Applying standards to internal data and client documents were deemed as quick wins to improving process
80% agreed that client risk profiling and the analysis across multiple data sources can be most improved - AI can improve KPI’s on False Positives, Client Risk, Automation & False Negatives.
While the appetite for AI & Machine Learning is increasing but firms need to develop effective risk controls pre-implementation
Often the end to end process is not questioned; firms need to look beyond the point tech, and define the use case for value
Illuminating anecdotes shared on how to make the business case for AI/ Tech. Business, AFC Analysts and Ops have different needs
Firms face a real skills gap in order to move from a traditional AFC approach to an intelligent-data led one. Where are the teachers?
60% of respondents had gone live with AI in at least one business use-case or were looking to transition to an AI-led operating model
AI & Anti-Financial Crime
Whether it is a judgement on the accuracy of a Client’s ID, an assessment of the level of money laundering risk they pose, or a decision on client documentation, AI has the potential to improve accuracy and speed in a variety of areas of the AFC and CLM process.
AI can help improve speed and accuracy of AFC client verification, risk profiling, screening and monitoring with a variety approaches. The two key ways AI can benefit AFC are:
- Process automation – AI can help firms in taking the minimum number of steps and the data required to assemble a complete KYC file, complete due diligence, and to assign a risk rating for a client
- Risk management – AI can help firms better understand and profile clients into micro-segments, enabling more accurate risk assessment, reducing the amount of false positives that firms have to process
Holistic examination of the underlying metadata assembled and challenging AI decisions will be necessary to prevent build up of risk and biases
Mass retraining will be necessary when AI becomes more integral to businesses
KYC / Customer Due Diligence (CDD)
Key challenge: How can anti-money laundering (AML) operations be improved through machine learning?
Firms’ KYC / CDD processes are hindered by high volumes of client documentation, the difficulty in validating clients’ identity and the significant level of compliance requirements
AI can link, enrich and enhance transactions, risk and customer data sets to create risk-intelligence allowing firms to better assess and predict clients’ risk rating dynamically and in real-time based on expected and ongoing behaviour - this improves both the risk assessment and also the speed of onboarding
AI can profile clients through the use of entity resolution which establishes confidence in the truth of the clients identity by matching them against their potential network generated by analysis of the initial data set provided by client
Better matches can be predicted by deriving additional data from existing and external data sources to further enhance scope & accuracy of client’s network
The result is a clear view of the client’s identity and relationships within the context of their environment underpinned by the transparent and traceable layers of probability generated by the underlying data set
To improve data quality, firms need to be able to set standards for their internal data and their client’s documentation
82% of respondents cited ‘Risk Analysis & Profiling’ as having the most opportunity for improvement through AI
If documentation is in a poor state, you've got to find something else to measure for risk – technology that provides additional context is valuable
Transaction Screening
Key pains faced by firms are the number of false positives (transactions flagged as risky that are subsequently found to be safe), the resulting workload in investigating them, as well as the volume of ‘false negatives’ (transactions that are flagged as risky, but released incorrectly)
AI can help improve the accuracy and efficiency of transaction and payment screening at a tactical and strategic level
Tactically, AI can reduce workload by carrying out the necessary checks and transactions analysis. AI can automate processes such as structuring of the transaction, verification of the transaction profile and discrepancy checks
Strategically, AI can reduce the volume of checks necessary in the first place by better assessing the client’s risk (i.e., reducing the number of high risk clients by 10% through better risk assessment reduces the volume of investigatory checks).
AI can assist in automating the corresponding investigative processes, which are currently often highly manual, email intensive with lots of to-and-fro.
A ‘White List’ of transactions allows much smoother processing of transactions compared to due diligence whenever a transaction is flagged
82% of respondents cited ‘Risk Analysis & Profiling’ as a key area that could be most improved by AI applications
Transaction Monitoring
Firms suffer from a high number of false positives and investigative overhead due to rules-based monitoring and coarse client segmentation
AI can help reduce the number of false positives and increase the efficiency of investigative work by allowing monitoring rules to target more granular types of clients (segments), updating the rules according to client’s behaviour, and intelligently informing investigators when alerts can be dispositioned.
AI can expand the list of features that you can segment clients on (e.g. does a retailer have an ATM on site?) and identify the hidden patterns that associate specific groups of clients (e.g., Client A, an exporter, is transacting with an entity type that other exporters do not). It can use a firm’s internal data sources and a variety of external data sources to create enriched data intelligence.
Reinforcement learning allows firms to adjust their own algorithms and rules for specific segments of clients and redefine those rules and thresholds to identify correlations and deviations, so different types of clients get treated differently according to their behaviour and investigative results
Survey Results
90% of respondents to Leading Point FM’s survey on AI and Anti-Financial Crime cited ‘Volume & Quality of Data’ as being one of the top 3 biggest challenges for CLM and AFC functions in 2019
82% of respondents to cited ‘Risk Analysis & Profiling’ as having the most opportunity for improvement through AI
60% of respondents had gone live with Artificial Intelligence in at least one business use case or were looking to transition to an AI-led operating model.
However, 40% were unclear on what solutions were available 60% of respondents cited ‘Immaturity of Technology’ or ‘Lack of Business Case’ as the biggest obstacle to adopting AI applications
Conclusion
To apply AI practically requires an understanding of the sweet spot between automation and assisting, leveraging human users’ knowledge and expertise
AI needs a well-defined use case to be successful as it can’t solve for all KYC problems at the same time. In order to deliver value, clarity on KPI’s that matter and reviewing AI considering the end-to-end business process is important.
Defining the core, minimal data set needed to support a business outcome, meet compliance requirements, and enable risk assessment will help firms make decisions on what existing data collection processes/ sources are needed, and where AI tech can support enrichment. It is possible to reduce data collection by 60-70% and significantly improve client digital journeys.
There are significant skills gaps in order to move from a traditional AFC op model to more intelligent-data AI led one. When AI becomes more integral to business, mass re-training will be necessary. So, where are the teachers?
The move from repetitive low value-added tasks to more intelligent-data based operating models. Industry collaborations & standards will help, but future competitive advantage will be a function of what are you doing with data that no one else is.
70% of respondents cited ‘Effort. Fatigue & False Positives’ as one of the top 3 biggest challenges for CLM and AFC functions in 2019?
More data isn’t always better. There is often a lot of redundant data that is gathered unnecessarily from the client.
Spotting suspicious activity via network analysis can be difficult if you only have visibility to one side of the transactions
If there's a problem worth solving, any large organisation will have at least six teams working on it – it comes down to the execution
LIBOR Transition - Preparation in the Face of Adversity
LIBOR TRANSITION IN CONTEXT
What is it? FCA will no longer seek require banks to submit quotes to the London Interbank Offered Rate (LIBOR) – LIBOR will be unsupported by regulators come 2021, and therefore, unreliable
Requirement: Firms need to transition away from LIBOR to alternative overnight risk-free rates (RFRs)
Challenge: Updating the risk and valuation processes to reflect RFR benchmarks and then reviewing the millions of legacy contracts to remove references to IBOR
Implementation timeline: Expected in Q4 2021
HOW LIBOR MAY IMPACT YOUR BUSINESS
Front office: New issuance and trading products to support capital, funding, liquidity, pricing, hedging
Finance & Treasury: Balance sheet valuation and accounting, asset, liability and liquidity management
Risk Management: New margin, exposure, counterparty risk models, VaR, time series, stress and sensitivities
Client outreach: Identification of in-scope contracts, client outreach and repapering to renegotiate current exposure
Change management: F2B data and platform changes to support all of the above
WHAT YOU NEED TO DO
Plug in to the relevant RFR and trade association working groups, understand internal advocacy positions vs. discussion outcomes
Assess, quantify and report LIBOR exposure across jurisdictions, businesses and products
Remediate data quality and align product taxonomies to ensure integrity of LIBOR exposure reporting
Evaluate potential changes to risk and valuation models; differences in accounting treatment under an alternative RFR regime
Define list of in-scope contracts and their repapering approach; prepare for client outreach
“[Firms should be] moving to contracts which do not rely on LIBOR and will not switch references rates at an unpredictable time”
Andrew Bailey, CEO,
Financial Conduct Authority (FCA)
“Identification of areas of no-regret spending is critical in this initial phase of delivery so as to give a head start to implementation”
Rajen Madan, CEO,
Leading Point FM
BENCHMARK TRANSITION KEY FACTS
- Market Exposure - Total IBOR market exposure >$370TN 80% represented by USD LIBOR & EURIBOR
- Tenor - The 3-month tenor by volume is the most widely referenced rate in all currencies (followed by the 6-month tenor)
- Derivatives - OTC and exchange traded derivatives represent > $300TN (80%) of products referencing IBORs
- Syndicated Loans - 97% of syndicated loans in the US market, with outstanding volume of approximately $3.4TN, reference USD LIBOR. 90% of syndicated loans in the euro market, with outstanding volume of approximately $535BN, reference EURIBOR
- Floating Rate Notes (FRNs) - 84% of FRNs inthe US market, with outstanding volume of approximately $1.5TN, reference USD LIBOR. 70% of FRNs in the euro market,with outstanding volume of approximately $2.6TN, reference EURIBOR
- Business Loans - 30%-50% of business loans in the US market, with outstanding volume of approximately $2.9TN, reference USD LIBOR. 60% of business loans in the euro market, with outstanding volume of approximately $5.8TN, reference EURIBOR
*(“IBOR Global Benchmark Survey 2018 Transition Roadmap”, ISDA, AFME, ICMA, SIFMA, SIFMA AM, February 2018)
Data Innovation, Uncovered
Leading Point Financial Markets recently partnered with selected tech companies to present innovative solutions to a panel of SMEs and an audience of FS senior execs and practitioners across 5 use-cases Leading Point is helping financial institutions with. The panel undertook a detailed discussion on the solutions’ feasibility within these use-cases, and their potential for firms, followed by a lively debate between Panellists and Attendees.
EXECUTIVE SUMMARY
“There is an opportunity to connect multiple innovation solutions to solve different, but related, business problems”
- 80% of data is relatively untapped in organisations. The more familiar the datasets, the better data can be used
- On average, an estimated £84 million (expected to be a gross underestimation) is wasted each year from increasing risk and delivery from policies and regulations
- Staying innovative, while staying true to privacy data is a fine line. Solutions exist in the marketplace to help
- Is there effective alignment between business and IT? Panellists insisted there is a significantly big gap, but using business architecture can be a successful bridge between the business and IT, by driving the right kinds of change
- There is a huge opportunity to blend these solutions to provide even more business benefits
CLIENT DATA LIFECYCLE (TAMR)
- Tamr uses machine learning to combine, consolidate and classify disparate data sources with potential to improve customer segmentation analytics
- To achieve the objective of a 360-degree view of the customer requires merging external datasets with internal in a appropriate and efficient manner, for example integrating ‘Politically Exposed Persons’ lists or sanctions ‘blacklists’
- Knowing what ‘good’ looks like is a key challenge. This requires defining your comfort level, in terms of precision and probability based approaches, versus the amount of resource required to achieve those levels
- Another challenge is convincing Compliance that machines are more accurate than individuals
- To convince the regulators, it is important to demonstrate that you are taking a ‘joined up’ approach across customers, transactions, etc. and the rationale behind that approach
LEGAL DOCS TO DATA (iManage)
- iManage locates, categorises & creates value from all your contractual content
- Firms hold a vast amount of legal information in unstructured formats - Classifying 30,000,000 litigation documents manually would take 27 years
- However, analysing this unstructured data and converting it to structured digital data allows firms to conduct analysis and repapering exercises with much more efficiency
- It is possible to a) codify regulations & obligations b) compare them as they change and c) link them to company policies & contracts – this enables complete traceability
- For example, you can use AI to identify parties, dates, clauses & conclusions held within ISDA contract forms, reports, loan application contracts, accounts and opinion pieces
DATA GOVERNANCE (Io-Tahoe)
- Io-Tahoe LLC is a provider of ‘smart’ data discovery solutions that go beyond traditional metadata and leverages machine learning and AI to look at implied critical and often unknown relationships within the data itself
- Io-Tahoe interrogates any structured/semi-structured data (both schema and underlying data) and identifies and classifies related data elements to determine their business criticality
- Pockets of previously-hidden sensitive data can be uncovered enabling better compliance to data protection regulations, such as GDPR
- Any and all data analysis is performed on copies of the data held wherever the information security teams of the client firms deems it safe
- Once data elements are understood, they can be defined & managed and used to drive data governance management processes
FINANCIAL CRIME (Ayasdi)
- Ayasdi augments the AML process with intelligent segmentation, typologies and alert triage. Their topological data analysis capabilities provide a formalised and repeatable way of applying hundreds of combinations of different machine learning algorithms to a data set to find out the relationships within that data
- For example, Ayasdi was used reason-based elements in predictive models to track, analyse and predict complaint patterns. over the next day, month and year.
- As a result, the transaction and customer data provided by a call centre was used effectively to reduce future complaints and generate business value
- Using Ayasdi, a major FS firm was able to achieve more than a 25% reduction in false positives and achieved savings of tens of millions of dollars - but there is still a lot more that can be done
DATA MONETISATION (Privitar)
- Privitar’s software solution allows the safe use of sensitive information enabling organisations to extract maximum data utility and economic benefit
- The sharp increase in data volume and usage in FS today has brought two competing dynamics: Data protection regulation aimed at protecting people from the misuse of their data and the absorption of data into tools/technologies such as machine learning
- However, as more data is made available, the harder it is to protect the privacy of the individual through data linkage
- Privitar’s tools are capable of removing a large amount of risk from this tricky area, and allow people to exchange data much more freely by anonymisation
- Privitar allows for open data for innovation and collaboration, whilst also acting in the best interest of customers’ privacy
SURVEY RESULTS
- Encouragingly, over 97% of participants who responded confirmed the five use cases presented were relevant to their respective organisations
- Nearly 50% of all participants who responded stated they would consider using the tech solutions presented
- 70% of responders believe their firms would be likely to adopt one of the solutions
- Only 10% of participants who responded believed the solutions were not relevant to their respective firms
- Approximately 30% of responders thought they would face difficulties in taking on a new solution
Reducing anti-financial crime risk through op model transformation at a tier 1 investment bank
“Leading Point have proven to be valued partners providing subject matter expertise and transformation delivery with sustained and consistent performance whilst becoming central to the Financial Crime Risk Management Transformation. They have been effective in providing advisory and practical implementation skills with an integrated approach bringing expertise in financial services and GRC (Governance, Risk and Compliance) functional and Fintech/Regtech technology domains."
Head of Anti-Financial Crime Design Authority @ Tier 1 Investment Bank
Rules of Data
On 24 October, it was reported that the Financial Conduct Authority launched an investigation into the US credit checking company Equifax; almost 700,000 Britons had their personal data misappropriated between mid-May and July this year. The FCA gave evidence on this matter to the Treasury Select Committee on 31 October because of the significant public interest. The FCA has the power to fine Equifax, or strip it of its right to operate in the UK, if it is found to have been negligent with its customers’ data. With European Union governments formally stating that cyber-attacks can be an ‘act of war,’ data protection cannot be taken seriously enough. The Equifax data breach is by no means a solitary data breach – several large organisations such as Dun & Bradstreet, Verifone, Whole Foods, Deloitte, DocuSign, Yahoo! are already part of the mix.
The Government is aligning domestic data legislation with the European Union in an effort at continuity, despite our plans to leave the EU. The Data Protection Bill, is proof that the Government seeks to keep the UK au courant with the newest data law of EU provenance.
The number of internet users is now close to 4 billion. Businesses continue to move their products and services online in order to service their customers. Data continues to grow exponentially and will persist in its travel far and wide – enabled by technology proliferation. The EU’s General Data Protection Regulation (‘GDPR’) has been precipitated by acute necessity. Companies need to review and revise their approach to privacy, security and governance of their data. A holistic, data protection framework is needed that is centred on the customer and encompasses their interactions, experience, sentiment, along with those of advocacy groups, shareholders, and regulators. This is a non trivial exercise and requires interventions at the mindset, policy, information governance & security and process levels, along with enabling technology.
Businesses are heading in the right direction with GDPR, but there is still a long way to go. Implementing this change with the right spirit is fundamental to building trust with customers and partners. Leading Point’s experience helping organisations with these requirements suggests that while significant compliance hurdles exist, a risk-based approach that focuses on five core areas, will be instrumental to success.
1. Give your customers control over their data – a mindset change
Bearing in mind the territorial scope of the GDPR – across the current 28 EU member states, plus, anyone dealing with the EU, most teams within organisations will benefit from the ethos behind the Regulation. A mindset shift from owning your customers’ data to stewarding your customers’ data is required. Give your customers control over their data. Any legal or natural person processing data must believe in the spirit of this sea change – the need
to assume responsibility for stewarding your customers’ data and to provide them with confidence in your processes. GDPR expands on the list of ‘rights’ each data subject is afforded: the right to be informed, the right to
access data records, the right to data erasure, to name a few. Tone at the top matters immensely.
2. Achieve Data Protection by Design
Which department is leading your organisation’s GDPR compliance efforts? A cross-functional team will help in deploying a holistic data protection framework. To start with, the focus must be on classification of the data, its
supply chain and its governance. Therefore, leveraging existing data management initiatives to embed data privacy requirements can really help in ‘data protection by design’. In practical terms, companies need a clear picture on: ‘what types of data do they hold on their customers;’ ‘which types of data is sensitive and requires enhanced security levels;’ ‘who has access to customers’ sensitive data;’ ‘where is this data processed and distributed;’ ‘how does it flow;’ ‘what is its quality;’ and ‘are their checks and controls in place around its flow and access’? The rules are more stringent now, as companies establish the depth of customer data – their interactions, experiences, sentiments – what impressions are left in an organisation’s data stores. The definition of personal data and its inherent breadth has been redefined – ‘Personal data shall be adequate, relevant and not excessive in relation to the purpose or purposes for which they are processed.’ And so the notion of data minimisation is born. We believe that while there are increasing numbers of quick-fix GDPR solutions in the market, achieving data protection goals is less about technology, and more about energising the organisation into becoming 100% data aware.
Building trust in your data will allow for effective process and controls for data protection, security and governance.
3. The Art of the Process
Focus must be on the ‘process’ exercise – visibility of customer journeys – which processes interact with customer data and the ensuing data lifecycle. Knowing which functions have client-facing processes and ensuring these are
adapted is called for. Threading through specific processes for data collection, data storage, data sharing, access requests and breaches is the focus. Having a command of what happens to personal data, who is involved in gathering it, and responding to Subject Access Requests is important, not least because you will have only a month to respond and cannot routinely charge the current £10. What steps to take in the event of a data breach, how to manage contracts which hold personal data: these are all explicit in the Regulation. For all data processors, we must double down on education and training – on policies, on data governance, on processes and new rules of data. This means highlighting a consistent approach to the different scenarios. Surely the best protection is a body of staff that is wholly informed?
4. Integrating data protection with a risk-based approach
By taking an inventory of obligations to customers via existing contracts and business agreements, organisations can start to manage their stated responsibilities linked to customer data and its management and use. This is a
quick-win.
Data classification and governance exercises will highlight the sensitivity, breadth and depth of data, the access and use of the data held. Data flow will highlight the data processors and third-parties and internal functions involved. Data quality will highlight where data management controls are required to be shored up. In turn, this will flag up priority remediation exercises on customer data.
The aforementioned ‘process’ exercise will highlight key customer-facing process changes, or a requirement to deploy specific data processes referenced by GDPR. Organisations can road-test these processes against the required process turn-around times. For example, data breaches must be reported within 72 hours, and as mentioned above, data subject access requests – one month. Involve your customer services team actively with data protection and security breach scenarios – this will build memory and promote mindset change.
The overarching governance in an organisation will be a key cog in the data protection ecosystem; the Regulation has duly led to the genesis of the Data Protection Officer. Enabling these responsibilities with existing data management governance responsibilities, and appointing data champions, can be an effective approach. Data protection is indisputably everyone’s responsibility, so the emphasis must be on organisational cooperation.
5. Cascading to Third Parties & a Cloud
Third party contracts and the framework that dictates how these are established, must wholeheartedly reflect any changes to the requisite data protection and security obligations. A compliance policy which standardises how third party contracts are established can also be a useful instrument. Data transference should be shored up with model contractual clauses, which allow all parties to clearly realise their responsibilities. We are alive to the persistent risk of cyber attacks, so it is crucial to remember that your data on the cloud is a business issue, as well as an IT issue. Are you fully apprised of where your business stores its data; on the premises, in the cloud, or both? The increasing trend to shift data and infrastructure to a public or private cloud no doubt presents an economic benefit and technology road map for some organisations. But make no mistake, organisations are accountable for their customer data content, its usage, and their security policy for cloud-based storage. Measures such as encryption, pseudonymisation and anonymisation will help, and should be employed as a matter of course, as well as remaining open to select technologies that help underpin cyber defence.
To conclude
When implementing change, evidence-based decision making shouldn’t be the only strategy; knowing which cogs in an organisation interlink cohesively in practice will greatly assist in a robust framework that threads through to
a mindset shift, policy, data, process and third parties. To reinforce an earlier perspective, data is only growing. So are data breaches and cyberattacks. The garnering of our data to feed algorithms and ‘machine learning’, borne
out of the Silicon Valley revolution, is leading to inevitable change in our lives, but we must strive for a democratic jurisdiction for our data. Organisations must give customers control of their data and the confidence in their data
management processes. Rather than penalty-based scaremongering, think of this as an opportunity to build your brand, to send a robust message to your customers and partners, demonstrating care and respect of their data.
To close, a soundbite from the Information Commissioner’s Office: ‘Data protection challenges arise not only from the volume of the data but from the ways in which it is generated, the propensity to find new uses for it, the complexity of the processing and the possibility of unexpected consequences for individuals.’
Leading Point Financial Markets brings compelling value in the intersection of Data, Compliance, Governance and Operating Model Change initiatives.